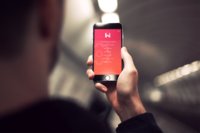
ISSUE
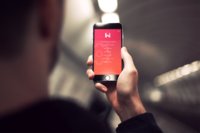
The Time Duration Calculator finds the number of days, minutes, hours and seconds between two given points in time.
The design of the calculator may vary slightly with this image.
timeanddate.com
A leap second will be added to Coordinated Universal Time (UTC) on December 31, 2016. This will help synchronize our watches with the Earth’s slowing rotation. more
The US state Indiana has 2 time zones: Central Time and Eastern Time. Daylight Saving Time (DST) has been used since 2006. more
The UK is not on Greenwich Mean Time (GMT) all year. During Daylight Saving Time the correct time zone is British Summer Time (BST). more
Most of the US state of Arizona does not use Daylight Saving Time (DST). The exception i
Hongli Gao (Ms.) gaohongl@msu.edu 4382 Okemos Road, Apt G214, Okemos, MI 48864 Tel: 517-703-6275 Teaching Portfolio Website: http://www.msu.edu/~gaohongl/index.html
Education
Michigan State University East Lansing, MI PhD Candidate in Mathematics (A.B.D.) Aug. 2009 – Sep. 2014 (Expected)
GPA: 3.9/4.0 Thesis: Minimization of a Non-Smooth Convex Functionals on an annulus domain Faculty Advisor: Baisheng YAN, Professor
Michigan State University East Lansing, MI Certification in Teaching College Mathematics Aug. 2012 – May. 2014 (Expected) – Teaching Portfolio: http://www.msu.edu/~gaohongl/index.html
Michigan State University East Lansing, MI M.Sc in Statistics Jan. – Dec. 2013 – GPA: 4.0/4.0 – Faculty Advisor: Dennis GILLILAND, Professor
Jilin University China M.Sc in Operational Research and Control Theory Sep. 2007 – June 2009 – Thesis: Waiting Time Phenomena of Solutions for Degenerate Parabolic Equations – Faculty Advisor: Jingxue YIN, Professor
Jilin University China B.Sc in Mathematics and Applied Mathematics Sep. 2003 – June 2007
Fellowships and Honors
Future Academic Scholars in Teaching (FAST) Fellowship East Lansing, MI Michigan State University 2012 – 2013
– One of the ten FAST fellows in 2012-2013 at Michigan State University – Acquisition of knowledge, skills, and competencies in teaching and learning in STEM disciplines
Graduate Teaching Assistant Award East Lansing, MI Department of Mathematics, Michigan State University 2013
– Two of over ninety teaching assistants in the Department of Mathematics
Academic Appointment
Instructor East Lansing, MI Michigan State University Aug. 2009 – present
Course Instructor
Courses: MTH 132 – Calculus I Spring 2013 MTH 133 – Calculus II Summer 2012, Fall 2012, Summer 2013 MTH 124 – A Survey of Calculus I Spring 2011, Summer 2011 MTH 103 – College Algebra Fall 2010 MTH 103 – College Algebra (online) Spring 2010, Summer 2010 MTH 1825 – Intermediate Algebra (online) Fall 2009 Responsibilities ∗ Independently develop syllabi and write the schedule of the classes ∗ Conduct classes, distribute the class notes and create in-class activities ∗ Implement the use of technology in lesson preparation and teaching ∗ Plan, set and evaluate quizzes and exams ∗ Hold office hours and identify the needs of individual students in their classes and help develop their potential ∗ Provide extra assistance to students with special needs, such as those with physical and mental disabilities
Recitation Instructor
Courses: MTH 133 – Calculus II Fall 2013 MTH 235 – Ordinary Differential Equations Fall 2011, Spring 2012 Responsibilities ∗ Present subject to students under the direction and guidance of instructor/supervisor ∗ Tutor and assist students individually or in small groups in order to help them master assignments and to reinforce learning concepts presented by teachers.
Academic Coach East Lansing, MI Charles Drew Science Scholars Program, Michigan State University Sep. 2013 – present
Courses: MTH 132 – Calculus I Fall 2013 MTH 133 – Calculus II Fall 2013 Responsibilities
Gao 2
∗ Coordinate students in Drew program and establish weekly meeting schedules ∗ Organize and prepare for instructional materials for lectures and offer coaching demonstrations meeting the needs of diverse students ∗ Offer advise to students on their learning process and help improve the students’ course performance
Supervisor East Lansing, MI Math Learning Center, Michigan State University Aug. 2012 – May 2013
Responsibilities ∗ Hold large review sessions for (about 100-200) students monthly ∗ Supervise and coordinate work of the monitor, proctor and 30 math tutors ∗ Suggest several constructive ideas to effectively improve the service quality and students’ satisfactory ∗ Observe other Calculus instructors/TAs’ teaching and offer suggestions
Instructor East Lansing, MI MSU Chinese School Sep. 2010 – present
Courses taught: Algebra I Fall 2010, Spring 2011, Fall 2011, Spring 2012, Fall 2013 Algebra II Fall 2012, Fall 2013 Geometry Spring 2013 Pre-Calculus Fall 2010, Spring 2011
Tutor East Lansing, MI Math Learning Center, Michigan State University Sep. 2009 – present
Courses: All undergraduate-level math classes
Other Professional Experience
QED Environmental System Inc. East Lansing, MI Data Analyst Jan. 2013 – May. 2013
• Performed data analysis on large (incomplete) data set and utilized the Artificial Neural Network (ANN) method to select variables
• Contributed to team effort by establishing a model of prediction and optimization of the target variables on MATLAB
• Provided suggestions on future data collecting and recommendations to optimize methane gas capture while maintaining safety guidelines for QED Environmental System Inc.
Consumers Energy Company East Lansing, MI Data Analyst & Model Developer Jan. 2012 – May. 2012
• Used a data mining tool to select the most possible variables from historical data
Gao 3
• Designed a program to estimate a customer’s probability of defaulting on a payment on R
• Provided several models used in industry for Consumers Energy Company to predict a customer’s likelihood of defaulting on a future payment
Research Interests and Projects
Research Interest
Partial Differential Equations, Calculus of Variations, Math education, Industrial Mathematics
Teaching As Research (TAR) Project, Michigan State University
How Does the Students’ “Demo” Effect a College-Level Calculus ClassSep. 2012 – Apr. 2013 Supervisor: Dr. Rique Campa Mentor: Dr. Milos Savic
Research in Industrial Mathematics, Michigan State University
Segmenting Customer Profiles by Modeling Default Probabilities Jan. 2012 – Apr. 2013 Supervisor: Dr. Peiru Wu Mentor: Dr. Albert Cohen
Optimization of Methane Gas at Landfills Jan. 2013 – Apr. 2013 Supervisor: Dr. Peiru Wu Mentor: Mr. Pavel Sikorskii
Conference & Professional Presentations
Joint Mathematics Meetings of the AMS and MAA Baltimore, MD Baltimore Convention Center Jan. 11 – 15, 2014
Future Academic Scholars in Teaching (FAST) Program Symposium East Lansing, MI Michigan State University Apr. 30 2013
Title: How Does the Students’ “Demo” Effect a College-Level Calculus Class
10th Michigan State University Student Math Conference East Lansing, MI Michigan State University Apr. 06 – 07, 2013
Title: Optimization of Methane Collection at Landfills
MSU Math Colloquium in Partial Differential Equations East Lansing, MI Department of Mathematics, Michigan State University Apr. 2012
Title: Reports on ”Asymptotic Convergence of Nonlinear Contraction Semigroups in Hilbert Space”
Gao 4
Institutional Service
Co-leader of Orientation for New Off-campus Instructors East Lansing, MI Michigan State University May 2013
– Led the teaching preparation component, helped the new off-campus instructors get prepared
Coordinator of MLC International Welcome Event East Lansing, MI Department of Mathematics, Michigan State University Sept. 2012
– Managed the entire event including preparation work, coordinated speakers and students
Skills And Qualifications
Languages: English (fluent), Chinese (native), French (Ph.D. language exam passed, reading only)
Computer skills: Matlab, R, SAS
PROFESSIONAL AFFILIATIONS
Society for Industrial and Applied Mathematics (SIAM)
American Mathematical Society (AMS)
Center for the Integration of Research, Teaching, and Learning (CIRTL)
References
Casim Abbas Professor, Associate Chair and Director of the Graduate Program Department of Mathematics Michigan State University (517) 353-4650 Email: abbas@math.msu.edumicigen 2012micigen 2012
Independently gave lectures, wrote and graded quizzes and exams, held office hours
By Eva L. Baker, Paul E. Barton, Linda Darling-Hammond, Edward Haertel, Helen F. Ladd, Robert L. Linn, Diane Ravitch, Richard Rothstein, Richard J. Shavelson, and Lorrie A. Shepard
Every classroom should have a well-educated, professional teacher, and school systems should recruit, prepare, and retain teachers who are qualified to do the job. Yet in practice, American public schools generally do a poor job of systematically developing and evaluating teachers.
Many policy makers have recently come to believe that this failure can be remedied by calculating the improvement in students’ scores on standardized tests in mathematics and reading, and then relying heavily on these calculations to evaluate, reward, and remove the teachers of these tested students.
While there are good reasons for concern about the current system of teacher evaluation, there are also good reasons to be concerned about claims that measuring teachers’ effectiveness largely by student test scores will lead to improved student achievement. If new laws or policies specifically require that teachers be fired if their students’ test scores do not rise by a certain amount, then more teachers might well be terminated than is now the case. But there is not strong evidence to indicate either that the departing teachers would actually be the weakest teachers, or that the departing teachers would be replaced by more effective ones. There is also little or no evidence for the claim that teachers will be more motivated to improve student learning if teachers are evaluated or monetarily rewarded for student test score gains.
A review of the technical evidence leads us to conclude that, although standardized test scores of students are one piece of information for school leaders to use to make judgments about teacher effectiveness, such scores should be only a part of an overall comprehensive evaluation. Some states are now considering plans that would give as much as 50% of the weight in teacher evaluation and compensation decisions to scores on existing tests of basic skills in math and reading. Based on the evidence, we consider this unwise. Any sound evaluation will necessarily involve a balancing of many factors that provide a more accurate view of what teachers in fact do in the classroom and how that contributes to student learning.
Recent statistical advances have made it possible to look at student achievement gains after adjusting for some student and school characteristics. These approaches that measure growth using “value-added modeling” (VAM) are fairer comparisons of teachers than judgments based on their students’ test scores at a single point in time or comparisons of student cohorts that involve different students at two points in time. VAM methods have also contributed to stronger analyses of school progress, program influences, and the validity of evaluation methods than were previously possible.
Nonetheless, there is broad agreement among statisticians, psychometricians, and economists that student test scores alone are not sufficiently reliable and valid indicators of teacher effectiveness to be used in high-stakes personnel decisions, even when the most sophisticated statistical applications such as value-added modeling are employed.
For a variety of reasons, analyses of VAM results have led researchers to doubt whether the methodology can accurately identify more and less effective teachers. VAM estimates have proven to be unstable across statistical models, years, and classes that teachers teach. One study found that across five large urban districts, among teachers who were ranked in the top 20% of effectiveness in the first year, fewer than a third were in that top group the next year, and another third moved all the way down to the bottom 40%. Another found that teachers’ effectiveness ratings in one year could only predict from 4% to 16% of the variation in such ratings in the following year. Thus, a teacher who appears to be very ineffective in one year might have a dramatically different result the following year. The same dramatic fluctuations were found for teachers ranked at the bottom in the first year of analysis. This runs counter to most people’s notions that the true quality of a teacher is likely to change very little over time and raises questions about whether what is measured is largely a “teacher effect” or the effect of a wide variety of other factors.
A study designed to test this question used VAM methods to assign effects to teachers after controlling for other factors, but applied the model backwards to see if credible results were obtained. Surprisingly, it found that students’ fifth grade teachers were good predictors of their fourth grade test scores. Inasmuch as a student’s later fifth grade teacher cannot possibly have influenced that student’s fourth grade performance, this curious result can only mean that VAM results are based on factors other than teachers’ actual effectiveness.
VAM’s instability can result from differences in the characteristics of students assigned to particular teachers in a particular year, from small samples of students (made even less representative in schools serving disadvantaged students by high rates of student mobility), from other influences on student learning both inside and outside school, and from tests that are poorly lined up with the curriculum teachers are expected to cover, or that do not measure the full range of achievement of students in the class.
For these and other reasons, the research community has cautioned against the heavy reliance on test scores, even when sophisticated VAM methods are used, for high stakes decisions such as pay, evaluation, or tenure. For instance, the Board on Testing and Assessment of the National Research Council of the National Academy of Sciences stated,
…VAM estimates of teacher effectiveness should not be used to make operational decisions because such estimates are far too unstable to be considered fair or reliable.
A review of VAM research from the Educational Testing Service’s Policy Information Center concluded,
VAM results should not serve as the sole or principal basis for making consequential decisions about teachers. There are many pitfalls to making causal attributions of teacher effectiveness on the basis of the kinds of data available from typical school districts. We still lack sufficient understanding of how seriously the different technical problems threaten the validity of such interpretations.
And RAND Corporation researchers reported that,
The estimates from VAM modeling of achievement will often be too imprecise to support some of the desired inferences…
and that
The research base is currently insufficient to support the use of VAM for high-stakes decisions about individual teachers or schools.
A number of factors have been found to have strong influences on student learning gains, aside from the teachers to whom their scores would be attached. These include the influences of students’ other teachers—both previous teachers and, in secondary schools, current teachers of other subjects—as well as tutors or instructional specialists, who have been found often to have very large influences on achievement gains. These factors also include school conditions—such as the quality of curriculum materials, specialist or tutoring supports, class size, and other factors that affect learning. Schools that have adopted pull-out, team teaching, or block scheduling practices will only inaccurately be able to isolate individual teacher “effects” for evaluation, pay, or disciplinary purposes.
Student test score gains are also strongly influenced by school attendance and a variety of out-of-school learning experiences at home, with peers, at museums and libraries, in summer programs, on-line, and in the community. Well-educated and supportive parents can help their children with homework and secure a wide variety of other advantages for them. Other children have parents who, for a variety of reasons, are unable to support their learning academically. Student test score gains are also influenced by family resources, student health, family mobility, and the influence of neighborhood peers and of classmates who may be relatively more advantaged or disadvantaged.
Teachers’ value-added evaluations in low-income communities can be further distorted by the summer learning loss their students experience between the time they are tested in the spring and the time they return to school in the fall. Research shows that summer gains and losses are quite substantial. A research summary concludes that while students overall lose an average of about one month in reading achievement over the summer, lower-income students lose significantly more, and middle-income students may actually gain in reading proficiency over the summer, creating a widening achievement gap. Indeed, researchers have found that three-fourths of schools identified as being in the bottom 20% of all schools, based on the scores of students during the school year, would not be so identified if differences in learning outside of school were taken into account. Similar conclusions apply to the bottom 5% of all schools.
For these and other reasons, even when methods are used to adjust statistically for student demographic factors and school differences, teachers have been found to receive lower “effectiveness” scores when they teach new English learners, special education students, and low-income students than when they teach more affluent and educationally advantaged students. The nonrandom assignment of students to classrooms and schools—and the wide variation in students’ experiences at home and at school—mean that teachers cannot be accurately judged against one another by their students’ test scores, even when efforts are made to control for student characteristics in statistical models.
Recognizing the technical and practical limitations of what test scores can accurately reflect, we conclude that changes in test scores should be used only as a modest part of a broader set of evidence about teacher practice.
Besides concerns about statistical methodology, other practical and policy considerations weigh against heavy reliance on student test scores to evaluate teachers. Research shows that an excessive focus on basic math and reading scores can lead to narrowing and over-simplifying the curriculum to only the subjects and formats that are tested, reducing the attention to science, history, the arts, civics, and foreign language, as well as to writing, research, and more complex problem-solving tasks.
Tying teacher evaluation and sanctions to test score results can discourage teachers from wanting to work in schools with the neediest students, while the large, unpredictable variation in the results and their perceived unfairness can undermine teacher morale. Surveys have found that teacher attrition and demoralization have been associated with test-based accountability efforts, particularly in high-need schools.
Individual teacher rewards based on comparative student test results can also create disincentives for teacher collaboration. Better schools are collaborative institutions where teachers work across classroom and grade-level boundaries toward the common goal of educating all children to their maximum potential. A school will be more effective if its teachers are more knowledgeable about all students and can coordinate efforts to meet students’ needs.
Some other approaches, with less reliance on test scores, have been found to improve teachers’ practice while identifying differences in teachers’ effectiveness. They use systematic observation protocols with well-developed, research-based criteria to examine teaching, including observations or videotapes of classroom practice, teacher interviews, and artifacts such as lesson plans, assignments, and samples of student work. Quite often, these approaches incorporate several ways of looking at student learning over time in relation to a teacher’s instruction.
Evaluation by competent supervisors and peers, employing such approaches, should form the foundation of teacher evaluation systems, with a supplemental role played by multiple measures of student learning gains that, where appropriate, could include test scores. Some districts have found ways to identify, improve, and as necessary, dismiss teachers using strategies like peer assistance and evaluation that offer intensive mentoring and review panels. These and other approaches should be the focus of experimentation by states and districts.
Adopting an invalid teacher evaluation system and tying it to rewards and sanctions is likely to lead to inaccurate personnel decisions and to demoralize teachers, causing talented teachers to avoid high-needs students and schools, or to leave the profession entirely, and discouraging potentially effective teachers from entering it. Legislatures should not mandate a test-based approach to teacher evaluation that is unproven and likely to harm not only teachers, but also the children they instruct.
Every classroom should have a well-educated, professional teacher. For that to happen, school systems should recruit, prepare, and retain teachers who are qualified to do the job. Once in the classroom, teachers should be evaluated on a regular basis in a fair and systematic way. Effective teachers should be retained, and those with remediable shortcomings should be guided and trained further. Ineffective teachers who do not improve should be removed.
In practice, American public schools generally do a poor job of systematically developing and evaluating teachers. School districts often fall short in efforts to improve the performance of less effective teachers, and failing that, of removing them. Principals typically have too broad a span of control (frequently supervising as many as 30 teachers), and too little time and training to do an adequate job of assessing and supporting teachers. Many principals are themselves unprepared to evaluate the teachers they supervise. Due process requirements in state law and union contracts are sometimes so cumbersome that terminating ineffective teachers can be quite difficult, except in the most extreme cases. In addition, some critics believe that typical teacher compensation systems provide teachers with insufficient incentives to improve their performance.
In response to these perceived failures of current teacher policies, the Obama administration encourages states to make greater use of students’ test results to determine a teacher’s pay and job tenure. Some advocates of this approach expect the provision of performance-based financial rewards to induce teachers to work harder and thereby increase their effectiveness in raising student achievement. Others expect that the apparent objectivity of test-based measures of teacher performance will permit the expeditious removal of ineffective teachers from the profession and will encourage less effective teachers to resign if their pay stagnates. Some believe that the prospect of higher pay for better performance will attract more effective teachers to the profession and that a flexible pay scale, based in part on test-based measures of effectiveness, will reduce the attrition of more qualified teachers whose commitment to teaching will be strengthened by the prospect of greater financial rewards for success.
Encouragement from the administration and pressure from advocates have already led some states to adopt laws that require greater reliance on student test scores in the evaluation, discipline, and compensation of teachers. Other states are considering doing so.
While there are many reasons for concern about the current system of teacher evaluation, there are also reasons to be skeptical of claims that measuring teachers’ effectiveness by student test scores will lead to the desired outcomes. To be sure, if new laws or district policies specifically require that teachers be fired if their students’ test scores do not rise by a certain amount or reach a certain threshold, then more teachers might well be terminated than is now the case. But there is no current evidence to indicate either that the departing teachers would actually be the weakest teachers, or that the departing teachers would be replaced by more effective ones. Nor is there empirical verification for the claim that teachers will improve student learning if teachers are evaluated based on test score gains or are monetarily rewarded for raising scores.
The limited existing indirect evidence on this point, which emerges from the country’s experience with the No Child Left Behind (NCLB) law, does not provide a very promising picture of the power of test-based accountability to improve student learning. NCLB has used student test scores to evaluate schools, with clear negative sanctions for schools (and, sometimes, their teachers) whose students fail to meet expected performance standards. We can judge the success (or failure) of this policy by examining results on the National Assessment of Educational Progress (NAEP), a federally administered test with low stakes, given to a small (but statistically representative) sample of students in each state.
The NCLB approach of test-based accountability promised to close achievement gaps, particularly for minority students. Yet although there has been some improvement in NAEP scores for African Americans since the implementation of NCLB, the rate of improvement was not much better in the post- than in the pre-NCLB period, and in half the available cases, it was worse. Scores rose at a much more rapid rate before NCLB in fourth grade math and in eighth grade reading, and rose faster after NCLB in fourth grade reading and slightly faster in eighth grade math. Furthermore, in fourth and eighth grade reading and math, white students’ annual achievement gains were lower after NCLB than before, in some cases considerably lower. Table 1 displays rates of NAEP test score improvement for African American and white students both before and after the enactment of NCLB. These data do not support the view that that test-based accountability increases learning gains.
Table 1 shows only simple annual rates of growth, without statistical controls. A recent careful econometric study of the causal effects of NCLB concluded that during the NCLB years, there were noticeable gains for students overall in fourth grade math achievement, smaller gains in eighth grade math achievement, but no gains at all in fourth or eighth grade reading achievement. The study did not compare pre- and post-NCLB gains. The study concludes, “The lack of any effect in reading, and the fact that the policy appears to have generated only modestly larger impacts among disadvantaged subgroups in math (and thus only made minimal headway in closing achievement gaps), suggests that, to date, the impact of NCLB has fallen short of its extraordinarily ambitious, eponymous goals.”1
Such findings provide little support for the view that test-based incentives for schools or individual teachers are likely to improve achievement, or for the expectation that such incentives for individual teachers will suffice to produce gains in student learning. As we show in what follows, research and experience indicate that approaches to teacher evaluation that rely heavily on test scores can lead to narrowing and over-simplifying the curriculum, and to misidentifying both successful and unsuccessful teachers. These and other problems can undermine teacher morale, as well as provide disincentives for teachers to take on the neediest students. When attached to individual merit pay plans, such approaches may also create disincentives for teacher collaboration. These negative effects can result both from the statistical and practical difficulties of evaluating teachers by their students’ test scores.
A second reason to be wary of evaluating teachers by their students’ test scores is that so much of the promotion of such approaches is based on a faulty analogy—the notion that this is how the private sector evaluates professional employees. In truth, although payment for professional employees in the private sector is sometimes related to various aspects of their performance, the measurement of this performance almost never depends on narrow quantitative measures analogous to test scores in education. Rather, private-sector managers almost always evaluate their professional and lower-management employees based on qualitative reviews by supervisors; quantitative indicators are used sparingly and in tandem with other evidence. Management experts warn against significant use of quantitative measures for making salary or bonus decisions.2 The national economic catastrophe that resulted from tying Wall Street employees’ compensation to short-term gains rather than to longer-term (but more difficult-to-measure) goals is a particularly stark example of a system design to be avoided.
Other human service sectors, public and private, have also experimented with rewarding professional employees by simple measures of performance, with comparably unfortunate results.3 In both the United States and Great Britain, governments have attempted to rank cardiac surgeons by their patients’ survival rates, only to find that they had created incentives for surgeons to turn away the sickest patients. When the U.S. Department of Labor rewarded local employment offices for their success in finding jobs for displaced workers, counselors shifted their efforts from training programs leading to good jobs, to more easily found unskilled jobs that might not endure, but that would inflate the counselors’ success data. The counselors also began to concentrate on those unemployed workers who were most able to find jobs on their own, diminishing their attention to those whom the employment programs were primarily designed to help.
A third reason for skepticism is that in practice, and especially in the current tight fiscal environment, performance rewards are likely to come mostly from the redistribution of already-appropriated teacher compensation funds, and thus are not likely to be accompanied by a significant increase in average teacher salaries (unless public funds are supplemented by substantial new money from foundations, as is currently the situation in Washington, D.C.). If performance rewards do not raise average teacher salaries, the potential for them to improve the average effectiveness of recruited teachers is limited and will result only if the more talented of prospective teachers are more likely than the less talented to accept the risks that come with an uncertain salary. Once again, there is no evidence on this point.
And finally, it is important for the public to recognize that the standardized tests now in use are not perfect, and do not provide unerring measurements of student achievement. Not only are they subject to errors of various kinds—we describe these in more detail below—but they are narrow measures of what students know and can do, relying largely on multiple-choice items that do not evaluate students’ communication skills, depth of knowledge and understanding, or critical thinking and performance abilities. These tests are unlike the more challenging open-ended examinations used in high-achieving nations in the world.{{4 }}Indeed, U.S. scores on international exams that assess more complex skills dropped from 2000 to 2006,{{5 }}even while state and local test scores were climbing, driven upward by the pressures of test-based accountability.
This seemingly paradoxical situation can occur because drilling students on narrow tests does not necessarily translate into broader skills that students will use outside of test-taking situations. Furthermore, educators can be incentivized by high-stakes testing to inflate test results. At the extreme, numerous cheating scandals have now raised questions about the validity of high-stakes student test scores. Without going that far, the now widespread practice of giving students intense preparation for state tests—often to the neglect of knowledge and skills that are important aspects of the curriculum but beyond what tests cover—has in many cases invalidated the tests as accurate measures of the broader domain of knowledge that the tests are supposed to measure. We see this phenomenon reflected in the continuing need for remedial courses in universities for high school graduates who scored well on standardized tests, yet still cannot read, write or calculate well enough for first-year college courses. As policy makers attach more incentives and sanctions to the tests, scores are more likely to increase without actually improving students’ broader knowledge and understanding.6
Statisticians, psychometricians, and economists who have studied the use of test scores for high-stakes teacher evaluation, including its most sophisticated form, value-added modeling (VAM), mostly concur that such use should be pursued only with great caution. Donald Rubin, a leading statistician in the area of causal inference, reviewed a range of leading VAM techniques and concluded:
We do not think that their analyses are estimating causal quantities, except under extreme and unrealistic assumptions.7
A research team at RAND has cautioned that:
The estimates from VAM modeling of achievement will often be too imprecise to support some of the desired inferences.8
and,
The research base is currently insufficient to support the use of VAM for high-stakes decisions about individual teachers or schools.9
Henry Braun, then of the Educational Testing Service, concluded in his review of VAM research:
VAM results should not serve as the sole or principal basis for making consequential decisions about teachers. There are many pitfalls to making causal attributions of teacher effectiveness on the basis of the kinds of data available from typical school districts. We still lack sufficient understanding of how seriously the different technical problems threaten the validity of such interpretations.10
In a letter to the Department of Education, commenting on the Department’s proposal to use student achievement to evaluate teachers, the Board on Testing and Assessment of the National Research Council of the National Academy of Sciences wrote:
…VAM estimates of teacher effectiveness should not be used to make operational decisions because such estimates are far too unstable to be considered fair or reliable.11
And a recent report of a workshop conducted jointly by the National Research Council and the National Academy of Education concluded:
Value-added methods involve complex statistical models applied to test data of varying quality. Accordingly, there are many technical challenges to ascertaining the degree to which the output of these models provides the desired estimates. Despite a substantial amount of research over the last decade and a half, overcoming these challenges has proven to be very difficult, and many questions remain unanswered…12
Among the concerns raised by researchers are the prospects that value-added methods can misidentify both successful and unsuccessful teachers and, because of their instability and failure to disentangle other influences on learning, can create confusion about the relative sources of influence on student achievement. If used for high-stakes purposes, such as individual personnel decisions or merit pay, extensive use of test-based metrics could create disincentives for teachers to take on the neediest students, to collaborate with one another, or even to stay in the profession.
Basing teacher evaluation primarily on student test scores does not accurately distinguish more from less effective teachers because even relatively sophisticated approaches cannot adequately address the full range of statistical problems that arise in estimating a teacher’s effectiveness. Efforts to address one statistical problem often introduce new ones. These challenges arise because of the influence of student socioeconomic advantage or disadvantage on learning, measurement error and instability, the nonrandom sorting of teachers across schools and of students to teachers in classrooms within schools, and the difficulty of disentangling the contributions of multiple teachers over time to students’ learning. As a result, reliance on student test scores for evaluating teachers is likely to misidentify many teachers as either poor or successful.
Social scientists have long recognized that student test scores are heavily influenced by socioeconomic factors such as parents’ education and home literacy environment, family resources, student health, family mobility, and the influence of neighborhood peers, and of classmates who may be relatively more advantaged or disadvantaged. Thus, teachers working in affluent suburban districts would almost always look more effective than teachers in urban districts if the achievement scores of their students were interpreted directly as a measure of effectiveness.13
New statistical techniques, called value-added modeling (VAM), are intended to resolve the problem of socio-economic (and other) differences by adjusting for students’ prior achievement and demographic characteristics (usually only their income-based eligibility for the subsidized lunch program, and their race or Hispanic ethnicity).14 These techniques measure the gains that students make and then compare these gains to those of students whose measured background characteristics and initial test scores were similar, concluding that those who made greater gains must have had more effective teachers.
Value-added approaches are a clear improvement over status test-score comparisons (that simply compare the average student scores of one teacher to the average student scores of another); over change measures (that simply compare the average student scores of a teacher in one year to her average student scores in the previous year); and over growth measures (that simply compare the average student scores of a teacher in one year to the same students’ scores when they were in an earlier grade the previous year).15
Status measures primarily reflect the higher or lower achievement with which students entered a teacher’s classroom at the beginning of the year rather than the contribution of the teacher in the current year. Change measures are flawed because they may reflect differences from one year to the next in the various characteristics of students in a teacher’s classroom, as well as other school or classroom-related variables (e.g., the quality of curriculum materials, specialist or tutoring supports, class size, and other factors that affect learning). Growth measures implicitly assume, without justification, that students who begin at different achievement levels should be expected to gain at the same rate, and that all gains are due solely to the individual teacher to whom student scores are attached; growth measures do not control for students’ socioeconomic advantages or disadvantages that may affect not only their initial levels but their learning rates.
Although value-added approaches improve over these other methods, the claim that they can “level the playing field” and provide reliable, valid, and fair comparisons of individual teachers is overstated. Even when student demographic characteristics are taken into account, the value-added measures are too unstable (i.e., vary widely) across time, across the classes that teachers teach, and across tests that are used to evaluate instruction, to be used for the high-stakes purposes of evaluating teachers.16
Because education is both a cumulative and a complex process, it is impossible fully to distinguish the influences of students’ other teachers as well as school conditions on their apparent learning, let alone their out-of-school learning experiences at home, with peers, at museums and libraries, in summer programs, on-line, and in the community.
No single teacher accounts for all of a student’s achievement. Prior teachers have lasting effects, for good or ill, on students’ later learning, and several current teachers can also interact to produce students’ knowledge and skills. For example, with VAM, the essay-writing a student learns from his history teacher may be credited to his English teacher, even if the English teacher assigns no writing; the mathematics a student learns in her physics class may be credited to her math teacher. Some students receive tutoring, as well as homework help from well-educated parents. Even among parents who are similarly well- or poorly educated, some will press their children to study and complete homework more than others. Class sizes vary both between and within schools, a factor influencing achievement growth, particularly for disadvantaged children in the early grades.17 In some schools, counselors or social workers are available to address serious behavior or family problems, and in others they are not. A teacher who works in a well-resourced school with specialist supports may appear to be more effective than one whose students do not receive these supports.18 Each of these resource differences may have a small impact on a teacher’s apparent effectiveness, but cumulatively they have greater significance.
Although value-added methods can support stronger inferences about the influences of schools and programs on student growth than less sophisticated approaches, the research reports cited above have consistently cautioned that the contributions of VAM are not sufficient to support high-stakes inferences about individual teachers. Despite the hopes of many, even the most highly developed value-added models fall short of their goal of adequately adjusting for the backgrounds of students and the context of teachers’ classrooms. And less sophisticated models do even less well. The difficulty arises largely because of the nonrandom sorting of teachers to students across schools, as well as the nonrandom sorting of students to teachers within schools.
Nonrandom sorting of teachers to students across schools: Some schools and districts have students who are more socioeconomically disadvantaged than others. Several studies show that VAM results are correlated with the socioeconomic characteristics of the students.19 This means that some of the biases that VAM was intended to correct may still be operating. Of course, it could also be that affluent schools or districts are able to recruit the best teachers. This possibility cannot be ruled out entirely, but some studies control for cross-school variability and at least one study has examined the same teachers with different populations of students, showing that these teachers consistently appeared to be more effective when they taught more academically advanced students, fewer English language learners, and fewer low-income students.20 This finding suggests that VAM cannot control completely for differences in students’ characteristics or starting points.21
Teachers who have chosen to teach in schools serving more affluent students may appear to be more effective simply because they have students with more home and school supports for their prior and current learning, and not because they are better teachers. Although VAM attempts to address the differences in student populations in different schools and classrooms by controlling statistically for students’ prior achievement and demographic characteristics, this “solution” assumes that the socioeconomic disadvantages that affect children’s test scores do not also affect the rates at which they show progress—or the validity with which traditional tests measure their learning gains (a particular issue for English language learners and students with disabilities).
Some policy makers assert that it should be easier for students at the bottom of the achievement distribution to make gains because they have more of a gap to overcome. This assumption is not confirmed by research. Indeed, it is just as reasonable to expect that “learning begets learning”: students at the top of the distribution could find it easier to make gains, because they have more knowledge and skills they can utilize to acquire additional knowledge and skills and, because they are independent learners, they may be able to learn as easily from less effective teachers as from more effective ones.
The pattern of results on any given test could also be affected by whether the test has a high “ceiling”—that is, whether there is considerable room at the top of the scale for tests to detect the growth of students who are already high-achievers—or whether it has a low “floor”—that is, whether skills are assessed along a sufficiently long continuum for low-achieving students’ abilities to be measured accurately in order to show gains that may occur below the grade-level standard.22
Furthermore, students who have fewer out-of-school supports for their learning have been found to experience significant summer learning loss between the time they leave school in June and the time they return in the fall. We discuss this problem in detail below. For now, suffice it to say that teachers who teach large numbers of low-income students will be noticeably disadvantaged in spring-to-spring test gain analyses, because their students will start the fall further behind than more affluent students who were scoring at the same level in the previous spring.
The most acceptable statistical method to address the problems arising from the non-random sorting of students across schools is to include indicator variables (so-called school fixed effects) for every school in the data set. This approach, however, limits the usefulness of the results because teachers can then be compared only to other teachers in the same school and not to other teachers throughout the district. For example, a teacher in a school with exceptionally talented teachers may not appear to add as much value to her students as others in the school, but if compared to all the teachers in the district, she might fall well above average. In any event, teacher effectiveness measures continue to be highly unstable, whether or not they are estimated using school fixed effects.23
Nonrandom sorting of students to teachers within schools: A comparable statistical problem arises for teachers within schools, in that teachers’ value-added scores are affected by differences in the types of students who happen to be in their classrooms. It is commonplace for teachers to report that this year they had a “better” or “worse” class than last, even if prior achievement or superficial socioeconomic characteristics are similar.
Statistical models cannot fully adjust for the fact that some teachers will have a disproportionate number of students who may be exceptionally difficult to teach (students with poorer attendance, who have become homeless, who have severe problems at home, who come into or leave the classroom during the year due to family moves, etc.) or whose scores on traditional tests are frequently not valid (e.g., those who have special education needs or who are English language learners). In any school, a grade cohort is too small to expect each of these many characteristics to be represented in the same proportion in each classroom.
Another recent study documents the consequences of students (in this case, apparently purposefully) not being randomly assigned to teachers within a school. It uses a VAM to assign effects to teachers after controlling for other factors, but applies the model backwards to see if credible results obtain. Surprisingly, it finds that students’ fifth grade teachers appear to be good predictors of students’ fourth grade test scores.24 Inasmuch as a student’s later fifth grade teacher cannot possibly have influenced that student’s fourth grade performance, this curious result can only mean that students are systematically grouped into fifth grade classrooms based on their fourth grade performance. For example, students who do well in fourth grade may tend to be assigned to one fifth grade teacher while those who do poorly are assigned to another. The usefulness of value-added modeling requires the assumption that teachers whose performance is being compared have classrooms with students of similar ability (or that the analyst has been able to control statistically for all the relevant characteristics of students that differ across classrooms). But in practice, teachers’ estimated value-added effect necessarily reflects in part the nonrandom differences between the students they are assigned and not just their own effectiveness.
Purposeful, nonrandom assignment of students to teachers can be a function of either good or bad educational policy. Some grouping schemes deliberately place more special education students in selected inclusion classrooms or organize separate classes for English language learners. Skilled principals often try to assign students with the greatest difficulties to teachers they consider more effective. Also, principals often attempt to make assignments that match students’ particular learning needs to the instructional strengths of individual teachers. Some teachers are more effective with students with particular characteristics, and principals with experience come to identify these variations and consider them in making classroom assignments.
In contrast, some less conscientious principals may purposefully assign students with the greatest difficulties to teachers who are inexperienced, perhaps to avoid conflict with senior staff who resist such assignments. Furthermore, traditional tracking often sorts students by prior achievement. Regardless of whether the distribution of students among classrooms is motivated by good or bad educational policy, it has the same effect on the integrity of VAM analyses: the nonrandom pattern makes it extremely difficult to make valid comparisons of the value-added of the various teachers within a school.
In sum, teachers’ value-added effects can be compared only where teachers have the same mix of struggling and successful students, something that almost never occurs, or when statistical measures of effectiveness fully adjust for the differing mix of students, something that is exceedingly hard to do.
Unlike school, district, and state test score results based on larger aggregations of students, individual classroom results are based on small numbers of students leading to much more dramatic year-to-year fluctuations. Even the most sophisticated analyses of student test score gains generate estimates of teacher quality that vary considerably from one year to the next. In addition to changes in the characteristics of students assigned to teachers, this is also partly due to the small number of students whose scores are relevant for particular teachers.
Small sample sizes can provide misleading results for many reasons. No student produces an identical score on tests given at different times. A student may do less well than her expected score on a specific test if she comes to school having had a bad night’s sleep, and may do better than her expected score if she comes to school exceptionally well-rested. A student who is not certain of the correct answers may make more lucky guesses on multiple-choice questions on one test, and more unlucky guesses on another. Researchers studying year-to-year fluctuations in teacher and school averages have also noted sources of variation that affect the entire group of students, especially the effects of particularly cooperative or particularly disruptive class members.
Analysts must average test scores over large numbers of students to get reasonably stable estimates of average learning. The larger the number of students in a tested group, the smaller will be the average error because positive errors will tend to cancel out negative errors. But the sampling error associated with small classes of, say, 20-30 students could well be too large to generate reliable results. Most teachers, particularly those teaching elementary or middle school students, do not teach enough students in any year for average test scores to be highly reliable.
In schools with high mobility, the number of these students with scores at more than one point in time, so that gains can be measured, is smaller still. When there are small numbers of test-takers, a few students who are distracted during the test, or who are having a “bad” day when tests are administered, can skew the average score considerably. Making matters worse, because most VAM techniques rely on growth calculations from one year to the next, each teacher’s value-added score is affected by the measurement error in two different tests. In this respect VAM results are even less reliable indicators of teacher contributions to learning than a single test score. VAM approaches incorporating multiple prior years of data suffer similar problems.
In addition to the size of the sample, a number of other factors also affect the magnitude of the errors that are likely to emerge from value-added models of teacher effectiveness. In a careful modeling exercise designed to account for the various factors, a recent study by researchers at Mathematica Policy Research, commissioned and published by the Institute of Education Sciences of the U.S. Department of Education, concludes that the errors are sufficiently large to lead to the misclassification of many teachers.25
The Mathematica models, which apply to teachers in the upper elementary grades, are based on two standard approaches to value-added modeling, with the key elements of each calibrated with data on typical test score gains, class sizes, and the number of teachers in a typical school or district. Specifically, the authors find that if the goal is to distinguish relatively high or relatively low performing teachers from those with average performance within a district, the error rate is about 26% when three years of data are used for each teacher. This means that in a typical performance measurement system, more than one in four teachers who are in fact teachers of average quality would be misclassified as either outstanding or poor teachers, and more than one in four teachers who should be singled out for special treatment would be misclassified as teachers of average quality. If only one year of data is available, the error rate increases to 36%. To reduce it to 12% would require 10 years of data for each teacher.
Despite the large magnitude of these error rates, the Mathematica researchers are careful to point out that the resulting misclassification of teachers that would emerge from value-added models is still most likely understated because their analysis focuses on imprecision error alone. The failure of policy makers to address some of the validity issues, such as those associated with the nonrandom sorting of students across schools, discussed above, would lead to even greater misclassification of teachers.
Measurement error also renders the estimates of teacher quality that emerge from value-added models highly unstable. Researchers have found that teachers’ effectiveness ratings differ from class to class, from year to year, and from test to test, even when these are within the same content area.26 Teachers also look very different in their measured effectiveness when different statistical methods are used.27 Teachers’ value-added scores and rankings are most unstable at the upper and lower ends of the scale, where they are most likely to be used to allocate performance pay or to dismiss teachers believed to be ineffective.28
Because of the range of influences on student learning, many studies have confirmed that estimates of teacher effectiveness are highly unstable. One study examining two consecutive years of data showed, for example, that across five large urban districts, among teachers who were ranked in the bottom 20% of effectiveness in the first year, fewer than a third were in that bottom group the next year, and another third moved all the way up to the top 40%. There was similar movement for teachers who were highly ranked in the first year. Among those who were ranked in the top 20% in the first year, only a third were similarly ranked a year later, while a comparable proportion had moved to the bottom 40%.29
Another study confirmed that big changes from one year to the next are quite likely, with year-to-year correlations of estimated teacher quality ranging from only 0.2 to 0.4.30 This means that only about 4% to 16% of the variation in a teacher’s value-added ranking in one year can be predicted from his or her rating in the previous year.
These patterns, which held true in every district and state under study, suggest that there is not a stable construct measured by value-added measures that can readily be called “teacher effectiveness.”
That a teacher who appears to be very effective (or ineffective) in one year might have a dramatically different result the following year, runs counter to most people’s notions that the true quality of a teacher is likely to change very little over time. Such instability from year to year renders single year estimates unsuitable for high-stakes decisions about teachers, and is likely to erode confidence both among teachers and among the public in the validity of the approach.
The problems of measurement error and other sources of year-to-year variability are especially serious because many policy makers are particularly concerned with removing ineffective teachers in schools serving the lowest-performing, disadvantaged students. Yet students in these schools tend to be more mobile than students in more affluent communities. In highly mobile communities, if two years of data are unavailable for many students, or if teachers are not to be held accountable for students who have been present for less than the full year, the sample is even smaller than the already small samples for a single typical teacher, and the problem of misestimation is exacerbated.
Yet the failure or inability to include data on mobile students also distorts estimates because, on average, more mobile students are likely to differ from less mobile students in other ways not accounted for by the model, so that the students with complete data are not representative of the class as a whole. Even if state data systems permit tracking of students who change schools, measured growth for these students will be distorted, and attributing their progress (or lack of progress) to different schools and teachers will be problematic.
If policy makers persist in attempting to use VAM to evaluate teachers serving highly mobile student populations, perverse consequences can result. Once teachers in schools or classrooms with more transient student populations understand that their VAM estimates will be based only on the subset of students for whom complete data are available and usable, they will have incentives to spend disproportionately more time with students who have prior-year data or who pass a longevity threshold, and less time with students who arrive mid-year and who may be more in need of individualized instruction. And such response to incentives is not unprecedented: an unintended incentive created by NCLB caused many schools and teachers to focus greater effort on children whose test scores were just below proficiency cutoffs and whose small improvements would have great consequences for describing a school’s progress, while paying less attention to children who were either far above or far below those cutoffs.31
As noted above, even in a more stable community, the number of students in a given teacher’s class is often too small to support reliable conclusions about teacher effectiveness. The most frequently proposed solution to this problem is to limit VAM to teachers who have been teaching for many years, so their performance can be estimated using multiple years of data, and so that instability in VAM measures over time can be averaged out. This statistical solution means that states or districts only beginning to implement appropriate data systems must wait several years for sufficient data to accumulate. More critically, the solution does not solve the problem of nonrandom assignment, and it necessarily excludes beginning teachers with insufficient historical data and teachers serving the most disadvantaged (and most mobile) populations, thus undermining the ability of the system to address the goals policy makers seek.
The statistical problems we have identified here are not of interest only to technical experts. Rather, they are directly relevant to policy makers and to the desirability of efforts to evaluate teachers by their students’ scores. To the extent that this policy results in the incorrect categorization of particular teachers, it can harm teacher morale and fail in its goal of changing behavior in desired directions.
For example, if teachers perceive the system to be generating incorrect or arbitrary evaluations, perhaps because the evaluation of a specific teacher varies widely from year to year for no explicable reason, teachers could well be demoralized, with adverse effects on their teaching and increased desire to leave the profession. In addition, if teachers see little or no relationship between what they are doing in the classroom and how they are evaluated, their incentives to improve their teaching will be weakened.
The statistical concerns we have described are accompanied by a number of practical problems of evaluating teachers based on student test scores on state tests.
Most secondary school teachers, all teachers in kindergarten, first, and second grades and some teachers in grades three through eight do not teach courses in which students are subject to external tests of the type needed to evaluate test score gains. And even in the grades where such gains could, in principle, be measured, tests are not designed to do so.
Value-added measurement of growth from one grade to the next should ideally utilize vertically scaled tests, which most states (including large states like New York and California) do not use. In order to be vertically scaled, tests must evaluate content that is measured along a continuum from year to year. Following an NCLB mandate, most states now use tests that measure grade-level standards only and, at the high school level, end-of-course examinations, neither of which are designed to measure such a continuum. These test design constraints make accurate vertical scaling extremely difficult. Without vertically scaled tests, VAM can estimate changes in the relative distribution, or ranking, of students from last year to this, but cannot do so across the full breadth of curriculum content in a particular course or grade level, because many topics are not covered in consecutive years. For example, if multiplication is taught in fourth but not in fifth grade, while fractions and decimals are taught in fifth but not in fourth grade, measuring math “growth” from fourth to fifth grade has little meaning if tests measure only the grade level expectations. Furthermore, the tests will not be able to evaluate student achievement and progress that occurs well below or above the grade level standards.
Similarly, if probability, but not algebra, is expected to be taught in seventh grade, but algebra and probability are both taught in eighth grade, it might be possible to measure growth in students’ knowledge of probability, but not in algebra. Teachers, however, vary in their skills. Some teachers might be relatively stronger in teaching probability, and others in teaching algebra. Overall, such teachers might be equally effective, but VAM would arbitrarily identify the former teacher as more effective, and the latter as less so. In addition, if probability is tested only in eighth grade, a student’s success may be attributed to the eighth grade teacher even if it is largely a function of instruction received from his seventh grade teacher. And finally, if high school students take end-of-course exams in biology, chemistry, and physics in different years, for example, there is no way to calculate gains on tests that measure entirely different content from year to year.
Thus, testing expert Daniel Koretz concludes that “because of the need for vertically scaled tests, value-added systems may be even more incomplete than some status or cohort-to-cohort systems.”32
It is often quite difficult to match particular students to individual teachers, even if data systems eventually permit such matching, and to unerringly attribute student achievement to a specific teacher. In some cases, students may be pulled out of classes for special programs or instruction, thereby altering the influence of classroom teachers. Some schools expect, and train, teachers of all subjects to integrate reading and writing instruction into their curricula. Many classes, especially those at the middle-school level, are team-taught in a language arts and history block or a science and math block, or in various other ways. In schools with certain kinds of block schedules, courses are taught for only a semester, or even in nine or 10 week rotations, giving students two to four teachers over the course of a year in a given class period, even without considering unplanned teacher turnover. Schools that have adopted pull-out, team teaching, or block scheduling practices will have additional difficulties in isolating individual teacher “effects” for pay or disciplinary purposes.
Similarly, NCLB requires low-scoring schools to offer extra tutoring to students, provided by the school district or contracted from an outside tutoring service. High quality tutoring can have a substantial effect on student achievement gains.33 If test scores subsequently improve, should a specific teacher or the tutoring service be given the credit?
Teachers should not be held responsible for learning gains or losses during the summer, as they would be if they were evaluated by spring-to-spring test scores. These summer gains and losses are quite substantial. Indeed, researchers have found that three-fourths of schools identified as being in the bottom 20% of all schools, based on the scores of students during the school year, would not be so identified if differences in learning outside of school were taken into account.34 Similar conclusions apply to the bottom 5% of all schools.35
Another recent study showed that two-thirds of the difference between the ninth grade test scores of high and low socioeconomic status students can be traced to summer learning differences over the elementary years.36 A research summary concluded that while students overall lose an average of about one month in reading achievement over the summer, lower-income students lose significantly more, and middle-income students may actually gain in reading proficiency over the summer, creating a widening achievement gap.37 Teachers who teach a greater share of lower-income students are disadvantaged by summer learning loss in estimates of their effectiveness that are calculated in terms of gains in their students’ test scores from the previous year.
To rectify obstacles to value-added measurement presented both by the absence of vertical scaling and by differences in summer learning, schools would have to measure student growth within a single school year, not from one year to the next. To do so, schools would have to administer high stakes tests twice a year, once in the fall and once in the spring.38 While this approach would be preferable in some ways to attempting to measure value-added from one year to the next, fall and spring testing would force schools to devote even more time to testing for accountability purposes, and would set up incentives for teachers to game the value-added measures. However commonplace it might be under current systems for teachers to respond rationally to incentives by artificially inflating end-of-year scores by drill, test preparation activities, or teaching to the test, it would be so much easier for teachers to inflate their value-added ratings by discouraging students’ high performance on a September test, if only by not making the same extraordinary efforts to boost scores in the fall that they make in the spring.
The need, mentioned above, to have test results ready early enough in the year to influence not only instruction but also teacher personnel decisions is inconsistent with fall to spring testing, because the two tests must be spaced far enough apart in the year to produce plausibly meaningful information about teacher effects. A test given late in the spring, with results not available until the summer, is too late for this purpose. Most teachers will already have had their contracts renewed and received their classroom assignments by this time.39
Although the various reasons to be skeptical about the use of student test scores to evaluate teachers, along with the many conceptual and practical limitations of empirical value added measures, might suffice by themselves to make one wary of the move to test-based evaluation of teachers, they take on even greater significance in light of the potential for large negative effects of such an approach.
Using test scores to evaluate teachers unfairly disadvantages teachers of the neediest students. Because of the inability of value-added methods to fully account for the differences in student characteristics and in school supports, as well as the effects of summer learning loss, teachers who teach students with the greatest educational needs will appear to be less effective than they are. This could lead to the inappropriate dismissal of teachers of low-income and minority students, as well as of students with special educational needs. The success of such teachers is not
accurately captured by relative value-added metrics, and the use of VAM to evaluate such teachers could exacerbate disincentives to teach students with high levels of need. Teachers are also likely to be aware of personal circumstances (a move, an illness, a divorce) that are likely to affect individual students’ learning gains but are not captured by value-added models. Within a school, teachers will have incentives to avoid working with such students likely to pull down their teacher effectiveness scores.
Narrowing of the curriculum to increase time on what is tested is another negative consequence of high-stakes uses of value-added measures for evaluating teachers. This narrowing takes the form both of reallocations of effort between the subject areas covered in a full grade-level curriculum, and of reallocations of effort within subject areas themselves.40
The tests most likely to be used in any test-based teacher evaluation program are those that are currently required under NCLB, or that will be required under its reauthorized version. The current law requires that all students take standardized tests in math and reading each year in grades three through eight, and once while in high school. Although NCLB also requires tests in general science, this subject is tested only once in the elementary and middle grades, and the law does not count the results of these tests in its identification of inadequate schools. In practice, therefore, evaluating teachers by their students’ test scores means evaluating teachers only by students’ basic math and/or reading skills, to the detriment of other knowledge, skills, and experiences that young people need to become effective participants in a democratic society and contributors to a productive economy.
Thus, for elementary (and some middle-school) teachers who are responsible for all (or most) curricular areas, evaluation by student test scores creates incentives to diminish instruction in history, the sciences, the arts, music, foreign language, health and physical education, civics, ethics and character, all of which we expect children to learn. Survey data confirm that even with the relatively mild school-wide sanctions for low test scores provided by NCLB, schools have diminished time devoted to curricular areas other than math and reading. This shift was most pronounced in districts where schools were most likely to face sanctions—districts with schools serving low-income and minority children.41 Such pressures to narrow the curriculum will certainly increase if sanctions for low test scores are toughened to include the loss of pay or employment for individual teachers.
Another kind of narrowing takes place within the math and reading instructional programs themselves. There are two reasons for this outcome.
First, it is less expensive to grade exams that include only, or primarily, multiple-choice questions, because such questions can be graded by machine inexpensively, without employing trained professional scorers. Machine grading is also faster, an increasingly necessary requirement if results are to be delivered in time to categorize schools for sanctions and interventions, make instructional changes, and notify families entitled to transfer out under the rules created by No Child Left Behind. And scores are also needed quickly if test results are to be used for timely teacher evaluation. (If teachers are found wanting, administrators should know this before designing staff development programs or renewing teacher contracts for the following school year.)
As a result, standardized annual exams, if usable for high-stakes teacher or school evaluation purposes, typically include no or very few extended-writing or problem-solving items, and therefore do not measure conceptual understanding, communication, scientific investigation, technology and real-world applications, or a host of other critically important skills. Not surprisingly, several states have eliminated or reduced the number of writing and problem-solving items from their standardized exams since the implementation of NCLB.42 Although some reasoning and other advanced skills can be tested with multiple-choice questions, most cannot be, so teachers who are evaluated by students’ scores on multiple-choice exams have incentives to teach only lower level, procedural skills that can easily be tested.
Second, an emphasis on test results for individual teachers exacerbates the well-documented incentives for teachers to focus on narrow test-taking skills, repetitive drill, and other undesirable instructional practices. In mathematics, a brief exam can only sample a few of the many topics that teachers are expected to cover in the course of a year.43 After the first few years of an exam’s use, teachers can anticipate which of these topics are more likely to appear, and focus their instruction on these likely-to-be-tested topics, to be learned in the format of common test questions. Although specific questions may vary from year to year, great variation in the format of test questions is not practical because the expense of developing and field-testing significantly different exams each year is too costly and would undermine statistical equating procedures used to ensure the comparability of tests from one year to the next. As a result, increasing scores on students’ mathematics exams may reflect, in part, greater skill by their teachers in predicting the topics and types of questions, if not necessarily the precise questions, likely to be covered by the exam. This practice is commonly called “teaching to the test.” It is a rational response to incentives and is not unlawful, provided teachers do not gain illicit access to specific forthcoming test questions and prepare students for them.
Such test preparation has become conventional in American education and is reported without embarrassment by educators. A recent New York Times report, for example, described how teachers prepare students for state high school history exams:
As at many schools…teachers and administrators …prepare students for the tests. They analyze tests from previous years, which are made public, looking for which topics are asked about again and again. They say, for example, that the history tests inevitably include several questions about industrialization and the causes of the two world wars.44
A teacher who prepares students for questions about the causes of the two world wars may not adequately be teaching students to understand the consequences of these wars, although both are important parts of a
history curriculum. Similarly, if teachers know they will be evaluated by their students’ scores on a test that predictably asks questions about triangles and rectangles, teachers skilled in preparing students for calculations involving these shapes may fail to devote much time to polygons, an equally important but somewhat more difficult topic in the overall math curriculum.
In English, state standards typically include skills such as learning how to use a library and select appropriate books, give an oral presentation, use multiple sources of information to research a question and prepare a written argument, or write a letter to the editor in response to a newspaper article. However, these standards are not generally tested, and teachers evaluated by student scores on standardized tests have little incentive to develop student skills in these areas.45
A different kind of narrowing also takes place in reading instruction. Reading proficiency includes the ability to interpret written words by placing them in the context of broader background knowledge.46 Because children come to school with such wide variation in their background knowledge, test developers attempt to avoid unfairness by developing standardized exams using short, highly simplified texts.47Test questions call for literal meaning – identifying the main idea, picking out details, getting events in the right order—but without requiring inferential or critical reading abilities that are an essential part of proficient reading. It is relatively easy for teachers to prepare students for such tests by drilling them in the mechanics of reading, but this behavior does not necessarily make them good readers.48 Children prepared for tests that sample only small parts of the curriculum and that focus excessively on mechanics are likely to learn test-taking skills in place of mathematical reasoning and reading for comprehension. Scores on such tests will then be “inflated,” because they suggest better mathematical and reading ability than is in fact the case.
We can confirm that some score inflation has systematically taken place because the improvement in test scores of students reported by states on their high-stakes tests used for NCLB or state accountability typically far exceeds the improvement in test scores in math and reading on the NAEP.49 Because no school can anticipate far in advance that it will be asked to participate in the NAEP sample, nor which students in the school will be tested, and because no consequences for the school or teachers follow from high or low NAEP scores, teachers have neither the ability nor the incentive to teach narrowly to expected test topics. In addition, because there is no time pressure to produce results with fast electronic scoring, NAEP can use a variety of question formats including multiple-choice, constructed response, and extended open-ended responses.50 NAEP also is able to sample many more topics from a grade’s usual curriculum because in any subject it assesses, NAEP uses several test booklets that cover different aspects of the curriculum, with overall results calculated by combining scores of students who have been given different booklets. Thus, when scores on state tests used for accountability rise rapidly (as has typically been the case), while scores on NAEP exams for the same subjects and grades rise slowly or not at all, we can be reasonably certain that instruction was focused on the fewer topics and item types covered by the state tests, while topics and formats not covered on state tests, but covered on NAEP, were shortchanged.51
Another confirmation of score inflation comes from the Programme for International Student Assessment (PISA), a set of exams given to samples of 15-year-old students in over 60 industrialized and developing nations. PISA is highly regarded because, like national exams in high-achieving nations, it does not rely largely upon multiple-choice items. Instead, it evaluates students’ communication and critical thinking skills, and their ability to demonstrate that they can use the skills they have learned. U.S. scores and rankings on the international PISA exams dropped from 2000 to 2006, even while state and local test scores were climbing, driven upward by the pressures of test-based accountability. The contrast confirms that drilling students for narrow tests such as those used for accountability purposes in the United States does not necessarily translate into broader skills that students will use outside of test-taking situations.
A number of U.S. experiments are underway to determine if offers to teachers of higher pay, conditional on their students having higher test scores in math and reading, actually lead to higher student test scores in these subjects. We await the results of these experiments with interest. Even if they show that monetary incentives for teachers lead to higher scores in reading and math, we will still not know whether the higher scores were achieved by superior instruction or by more drill and test preparation, and whether the students of these teachers would perform equally well on tests for which they did not have specific preparation. Until such questions have been explored, we should be cautious about claims that experiments prove the value of pay-for-performance plans.
Better schools are collaborative institutions where teachers work across classroom and grade-level boundaries towards the common goal of educating all children to their maximum potential.52 A school will be more effective if its teachers are more knowledgeable about all students and can coordinate efforts to meet students’ needs. Collaborative work among teachers with different levels and areas of skill and different types of experience can capitalize on the strengths of some, compensate for the weaknesses of others, increase shared knowledge and skill, and thus increase their school’s overall professional capacity.
In one recent study, economists found that peer learning among small groups of teachers was the most powerful predictor of improved student achievement over time.{{53 }}Another recent study found that students achieve more in mathematics and reading when they attend schools characterized by higher levels of teacher collaboration for school improvement.54 To the extent that teachers are given incentives to pursue individual monetary rewards by posting greater test score gains than their peers, teachers may also have incentives to cease collaborating. Their interest becomes self-interest, not the interest of students, and their instructional strategies may distort and undermine their school’s broader goals.55
To enhance productive collaboration among all of a school’s staff for the purpose of raising overall student scores, group (school-wide) incentives are preferred to incentives that attempt to distinguish among teachers.
Individual incentives, even if they could be based on accurate signals from student test scores, would be unlikely to have a positive impact on overall student achievement for another reason. Except at the very bottom of the teacher quality distribution where test-based evaluation could result in termination, individual incentives will have little impact on teachers who are aware they are less effective (and who therefore expect they will have little chance of getting a bonus) or teachers who are aware they are stronger (and who therefore expect to get a bonus without additional effort). Studies in fields outside education have also documented that when incentive systems require employees to compete with one another for a fixed pot of monetary reward, collaboration declines and client outcomes suffer.56 On the other hand, with group incentives, everyone has a stronger incentive to be productive and to help others to be productive as well.57
A commonplace objection to a group incentive system is that it permits free riding—teachers who share in rewards without contributing additional effort. If the main goal, however, is student welfare, group incentives are still preferred, even if some free-riding were to occur.
Group incentives also avoid some of the problems of statistical instability we noted above: because a full school generates a larger sample of students than an individual classroom. The measurement of average achievement for all of a school’s students is, though still not perfectly reliable, more stable than measurement of achievement of students attributable to a specific teacher.
Yet group incentives, however preferable to individual incentives, retain other problems characteristic of individual incentives. We noted above that an individual incentive system that rewards teachers for their students’ mathematics and reading scores can result in narrowing the curriculum, both by reducing attention paid to non-tested curricular areas, and by focusing attention on the specific math and reading topics and skills most likely to be tested. A group incentive system can exacerbate this narrowing, if teachers press their colleagues to concentrate effort on those activities most likely to result in higher test scores and thus in group bonuses.
Pressure to raise student test scores, to the exclusion of other important goals, can demoralize good teachers and, in some cases, provoke them to leave the profession entirely.
Recent survey data reveal that accountability pressures are associated with higher attrition and reduced morale, especially among teachers in high-need schools.58Although such survey data are limited, anecdotes abound regarding the demoralization of apparently dedicated and talented teachers, as test-based accountability intensifies. Here, we reproduce two such stories, one from a St. Louis and another from a Los Angeles teacher:
No Child Left Behind has completely destroyed everything I ever worked for… We now have an enforced 90-minute reading block. Before, we always had that much reading in our schedule, but the difference now is that it’s 90 minutes of uninterrupted time. It’s impossible to schedule a lot of the things that we had been able to do before… If you take 90 minutes of time, and say no kids can come out at that time, you can’t fit the drama, band, and other specialized programs in… There is a ridiculous emphasis on fluency—reading is now about who can talk the fastest. Even the gifted kids don’t read for meaning; they just go as fast as they possibly can. Their vocabulary is nothing like it used to be. We used to do Shakespeare, and half the words were unknown, but they could figure it out from the context. They are now very focused on phonics of the words and the mechanics of the words, even the very bright kids are… Teachers feel isolated. It used to be different. There was more team teaching. They would say, “Can you take so-and-so for reading because he is lower?” That’s not happening… Teachers are as frustrated as I’ve ever seen them. The kids haven’t stopped wetting pants, or coming to school with no socks, or having arguments and fights at recess. They haven’t stopped doing what children do but the teachers don’t have time to deal with it. They don’t have time to talk to their class, and help the children figure out how to resolve things without violence. Teachable moments to help the schools and children function are gone. But the kids need this kind of teaching, especially inner-city kids and especially at the elementary levels.59
and,
[T]he pressure became so intense that we had to show how every single lesson we taught connected to a standard that was going to be tested. This meant that art, music, and even science and social studies were not a priority and were hardly ever taught. We were forced to spend ninety percent of the instructional time on reading and math. This made teaching boring for me and was a huge part of why I decided to leave the profession.60
If these anecdotes reflect the feelings of good teachers, then analysis of student test scores may distinguish teachers who are more able to raise test scores, but encourage teachers who are truly more effective to leave the profession.
Used with caution, value-added modeling can add useful information to comprehensive analyses of student progress and can help support stronger inferences about the influences of teachers, schools, and programs on student growth.
We began by noting that some advocates of using student test scores for teacher evaluation believe that doing so will make it easier to dismiss ineffective teachers. However, because of the broad agreement by technical experts that student test scores alone are not a sufficiently reliable or valid indicator of teacher effectiveness, any school district that bases a teacher’s dismissal on her students’ test scores is likely to face the prospect of drawn-out and expensive arbitration and/or litigation in which experts will be called to testify, making the district unlikely to prevail. The problem that advocates had hoped to solve will remain, and could perhaps be exacerbated.
There is simply no shortcut to the identification and removal of ineffective teachers. It must surely be done, but such actions will unlikely be successful if they are based on over-reliance on student test scores whose flaws can so easily provide the basis for successful challenges to any personnel action. Districts seeking to remove ineffective teachers must invest the time and resources in a comprehensive approach to evaluation that incorporates concrete steps for the improvement of teacher performance based on professional standards of instructional practice, and unambiguous evidence for dismissal, if improvements do not occur.
Some policy makers, acknowledging the inability fairly to identify effective or ineffective teachers by their students’ test scores, have suggested that low test scores (or value-added estimates) should be a “trigger” that invites further investigation. Although this approach seems to allow for multiple means of evaluation, in reality 100% of the weight in the trigger is test scores. Thus, all the incentives to distort instruction will be preserved to avoid identification by the trigger, and other means of evaluation will enter the system only after it is too late to avoid these distortions.
While those who evaluate teachers could take student test scores over time into account, they should be fully aware of their limitations, and such scores should be only one element among many considered in teacher profiles. Some states are now considering plans that would give as much as 50% of the weight in teacher evaluation and compensation decisions to scores on existing poor-quality tests of basic skills in math and reading. Based on the evidence we have reviewed above, we consider this unwise. If the quality, coverage, and design of standardized tests were to improve, some concerns would be addressed, but the serious problems of attribution and nonrandom assignment of students, as well as the practical problems described above, would still argue for serious limits on the use of test scores for teacher evaluation.
Although some advocates argue that admittedly flawed value-added measures are preferred to existing cumbersome measures for identifying, remediating, or dismissing ineffective teachers, this argument creates a false dichotomy. It implies there are only two options for evaluating teachers—the ineffectual current system or the deeply flawed test-based system.
Yet there are many alternatives that should be the subject of experiments. The Department of Education should actively encourage states to experiment with a range of approaches that differ in the ways in which they evaluate teacher practice and examine teachers’ contributions to student learning. These experiments should all be fully evaluated.
There is no perfect way to evaluate teachers. However, progress has been made over the last two decades in developing standards-based evaluations of teaching practice, and research has found that the use of such evaluations by some districts has not only provided more useful evidence about teaching practice, but has also been associated with student achievement gains and has helped teachers improve their practice and effectiveness.61 Structured performance assessments of teachers like those offered by the National Board for Professional Teaching Standards and the beginning teacher assessment systems in Connecticut and California have also been found to predict teacher’s effectiveness on value-added measures and to support teacher learning.62
These systems for observing teachers’ classroom practice are based on professional teaching standards grounded in research on teaching and learning. They use systematic observation protocols with well-developed, research-based criteria to examine teaching, including observations or videotapes of classroom practice, teacher interviews, and artifacts such as lesson plans, assignments, and samples of student work. Quite often, these approaches incorporate several ways of looking at student learning over time in relation to the teacher’s instruction.
Evaluation by competent supervisors and peers, employing such approaches, should form the foundation of teacher evaluation systems, with a supplemental role played by multiple measures of student learning gains that, where appropriate, should include test scores. Given the importance of teachers’ collective efforts to improve overall student achievement in a school, an additional component of documenting practice and outcomes should focus on the effectiveness of teacher participation in teams and the contributions they make to school-wide improvement, through work in curriculum development, sharing practices and materials, peer coaching and reciprocal observation, and collegial work with students.
In some districts, peer assistance and review programs—using standards-based evaluations that incorporate evidence of student learning, supported by expert teachers who can offer intensive assistance, and panels of administrators and teachers that oversee personnel decisions—have been successful in coaching teachers, identifying teachers for intervention, providing them assistance, and efficiently counseling out those who do not improve.63 In others, comprehensive systems have been developed for examining teacher performance in concert with evidence about outcomes for purposes of personnel decision making and compensation.64
Given the range of measures currently available for teacher evaluation, and the need for research about their effective implementation and consequences, legislatures should avoid imposing mandated solutions to the complex problem of identifying more and less effective teachers. School districts should be given freedom to experiment, and professional organizations should assume greater responsibility for developing standards of evaluation that districts can use. Such work, which must be performed by professional experts, should not be pre-empted by political institutions acting without evidence. The rule followed by any reformer of public schools should be: “First, do no harm.”
As is the case in every profession that requires complex practice and judgments, precision and perfection in the evaluation of teachers will never be possible. Evaluators may find it useful to take student test score information into account in their evaluations of teachers, provided such information is embedded in a more comprehensive approach. What is now necessary is a comprehensive system that gives teachers the guidance and feedback, supportive leadership, and working conditions to improve their performance, and that permits schools to remove persistently ineffective teachers without distorting the entire instructional program by imposing a flawed system of standardized quantification of teacher quality.
1. Dee and Jacob 2009, p. 36.
2. Rothstein, Jacobsen, and Wilder 2008, pp. 93-96.
3. Jauhar 2008; Rothstein, Jacobsen, and Wilder 2008, pp. 83-93.
4. Darling-Hammond 2010.
5. Baldi et al. 2007.
6. For a further discussion, see Ravitch 2010, Chapter 6.
7. Rubin, Stuart, and Zanutto 2004, p. 113
8. McCaffrey et al. 2004, p. 96.
9. McCaffrey et al. 2003, p. xx.
10. Braun 2005, p. 17.
11. BOTA 2009.
12. Braun, Chudowsky, and Koenig, 2010, p. vii.
13. Some policy makers seek to minimize these realities by citing teachers or schools who achieve exceptional results with disadvantaged students. Even where these accounts are true, they only demonstrate that more effective teachers and schools achieve better results, on average, with disadvantaged students than less effective teachers and schools achieve; they do not demonstrate that more effective teachers and schools achieve average results for disadvantaged students that are typical for advantaged students.
14. In rare cases, more complex controls are added to account for the influence of peers (i.e., the proportion of other students in a class who have similar characteristics) or the competence of the school’s principal and other leadership.
15. This taxonomy is suggested by Braun, Chudowsky, and Koenig 2010, pp. 3ff.
16. Rothstein 2010; Newton et al. forthcoming; Lockwood et al. 2007; Sass 2008.
17. Krueger 2003; Mosteller 1995; Glass et al. 1982.
18. For example, studies have found the effects of one-on-one or small group tutoring, generally conducted in pull-out sessions or after school by someone other than the classroom teacher, can be quite substantial. A meta-analysis (Cohen, Kulik, and Kulik 1982) of 52 tutoring studies reported that tutored students outperformed their classroom controls by a substantial average effect size of .40. Bloom (1984) noted that the average tutored student registered large gains of about 2 standard deviations above the average of a control class.
19. Newton et al., forthcoming.
20. Newton et al., forthcoming.
21. McCaffrey et al. (2004, p. 67) likewise conclude that “student characteristics are likely to confound estimated teacher effects when schools serve distinctly different populations.”
22. Poor measurement of the lowest achieving students has been exacerbated under NCLB by the policy of requiring alignment of tests to grade-level standards. If tests are too difficult, or if they are not aligned to the content students are actually learning, then they will not reflect actual learning gains.
23. Newton et al., forthcoming; Sass 2008; Schochet and Chiang 2010; Koedel and Betts 2007.
24. Rothstein 2010.
25. Schochet and Chiang 2010.
26. Sass 2008; Lockwood et al. 2007; Newton et al., forthcoming.
27. Newton et al., forthcoming; Rothstein 2010.
28. Braun 2005.
29. Sass 2008, citing Koedel and Betts 2007; McCaffrey et al. 2009. For similar findings, see Newton et al., forthcoming.
30. McCaffrey et al. 2009.
31. Diamond and Cooper 2007.
32. Koretz 2008b, p. 39.
33. See endnote 19, above, for citations to research on the impact of tutoring.
34. Downey, von Hippel, and Hughes 2008.
35. Heller, Downey, and von Hippel, forthcoming.
36. Alexander, Entwisle, and Olson 2007.
37. Cooper et al. 1996.
38. Although fall-to-spring testing ameliorates the vertical scaling problems, it does not eliminate them. Just as many topics are not taught continuously from one grade to another, so are many topics not taught continuously from fall to spring. During the course of a year, students are expected to acquire new knowledge and skills, some of which build on those from the beginning of the year, and some of which do not.
39. To get timely results, Colorado administers its standardized testing in March. Florida gave its writing test last year in mid-February and its reading, mathematics, and science tests in mid-March. Illinois did its accountability testing this year at the beginning of March. Texas has scheduled its testing to begin next year on March 1. Advocates of evaluating teachers by students’ fall-to-spring growth have not explained how, within reasonable budgetary constraints, all spring testing can be moved close to the end of the school year.
40. This formulation of the distinction has been suggested by Koretz 2008a.
41. McMurrer 2007; McMurrer 2008.
42. GAO 2009, p. 19.
43. For a discussion of curriculum sampling in tests, see Koretz 2008a, especially Chapter 2.
44. Medina 2010.
45. This argument has recently been developed in Hemphill and Nauer et al. 2010.
46. Hirsch 2006; Hirsch and Pondiscio 2010.
47. For discussion of these practices, see Ravitch 2003.
48. There is a well-known decline in relative test scores for low-income and minority students that begins at or just after the fourth grade, when more complex inferential skills and deeper background knowledge begin to play a somewhat larger, though still small role in standardized tests. Children who are enabled to do well by drilling the mechanics of decoding and simple, literal interpretation often do more poorly on tests in middle school and high school because they have neither the background knowledge nor the interpretive skills for the tasks they later confront. As the grade levels increase, gaming the exams by test prep becomes harder, though not impossible, if instruction begins to provide solid background knowledge in content areas and inferential skills. This is why accounts of large gains from test prep drill mostly concern elementary schools.
49. Lee 2006.
50. An example of a “constructed response” item might be a math problem for which a student must provide the correct answer and demonstrate the procedures for solving, without being given alternative correct and incorrect answers from which to choose. An example of an “open-ended response” might be a short essay for which there is no single correct answer, but in which the student must demonstrate insight, creativity, or reasoning ability.
51. Although less so than state standardized tests, even NAEP suffers from an excessive focus on “content-neutral” procedural skills, so the faster growth of state test scores relative to NAEP scores may understate the score inflation that has taken place. For further discussion of the attempt to make NAEP content-neutral, see Ravitch 2003.
52. Bryk and Schneider 2002; Neal 2009, pp. 160-162.
53. Jackson and Bruegmann 2009.
54. Goddard, Goddard, and Tschannen-Moran 2007.
55. Incentives could also operate in the opposite direction. Fifth grade teachers being evaluated by their students’ test scores might have a greater interest in pressing fourth grade teachers to better prepare their students for fifth grade. There is no way, however, to adjust statistically for a teacher’s ability to pressure other instructors in estimating the teacher’s effectiveness in raising her own students’ test scores.
56. See, for example, Lazear 1989.
57. Anh 2009.
58. Feng, Figlio, and Sass 2010; Finnigan and Gross 2007.
59. Rothstein, Jacobsen, and Wilder 2008, 189-190.
60. Rothstein, Jacobsen, and Wilder 2008, 50.
61. Milanowski, Kimball, and White 2004.
62. See for example, Bond et al. 2000; Cavaluzzo 2004; Goldhaber and Anthony 2004; Smith et al. 2005; Vandevoort, Amrein-Beardsley, and Berliner 2004; Wilson and Hallam 2006.
63. Darling-Hammond 2009; Van Lier 2008.
64. Denver’s Pro-comp system, Arizona’s Career Ladder, and the Teacher Advancement Program are illustrative. See for example, Solomon et al. 2007; Packard and Dereshiwsky 1991.
Ahn, Tom. 2009. “The Missing Link: Estimating the Impact of Incentives on Effort and Effort on Production Using Teacher Accountability Legislation.” Unpublished paper from thomas.ahn@uky.edu, September 27.
Alexander, Karl L., Doris R. Entwisle, and Linda Steffel Olson. 1972. Lasting consequences of the summer learning gap. American Sociological Review, 72: 167-180.
Baldi, Stéphane, et al. (Ying Jin, Melanie Skemer, Patricia J. Green, and Deborah Herget). 2007. Highlights From PISA 2006: Performance of U.S. 15-Year-Old Students in Science and Mathematics Literacy in an International Context. (NCES 2008–016). National Center for Education Statistics, Institute of Education Sciences, U.S. Department of Education. Washington, DC. http://nces.ed.gov/pubs2008/2008016.pdf. See also: PISA on line. OECD Programme for International Student Assessment. http://www.pisa.oecd.org/
Bloom, Benjamin S. 1984. The 2 sigma problem: The search for methods of group instruction as effective as one-to-one tutoring. Educational Researcher, 13 (6): 4–16.
Bond, Lloyd, et al. (Tracy Smith, Wanda K. Baker, and John A. Hattie). 2000. The Certification System of the National Board for Professional Teaching Standards: A Construct and Consequential Validity Study. Greensboro, N.C.: Center for Educational Research and Evaluation. http://www.nbpts.org/UserFiles/File/validity_1_-_UNC_Greebsboro_D_-_Bond.pdf
BOTA (Board on Testing and Assessment, Division of Behavioral and Social Sciences and Education, National Academy of Sciences). 2009. “Letter Report to the U.S. Department of Education on the Race to the Top Fund.” October 5. http://books.nap.edu/openbook.php?record_id=12780&page=1 (and ff)
Braun Henry. 2005. Using Student Progress to Evaluate Teachers: A Primer on Value-Added Models. Princeton, N.J.: Educational Testing Service. http://www.ets.org/Media/Research/pdf/PICVAM.pdf
Braun, Henry, Naomi Chudowsky, and Judith Koenig, Editors. 2010. Getting Value Out of Value-Added: Report of a Workshop. Committee on Value-Added Methodology for Instructional Improvement, Program Evaluation, and Accountability; National Research Council. http://www.nap.edu/catalog/12820.html
Bryk, Anthony S., and Barbara Schneider. 2002. Trust in Schools. A Core Resource for Improvement. New York: Russell Sage Foundation.
Cavaluzzo, Linda. 2004. Is National Board Certification an Effective Signal of Teacher Quality? (National Science Foundation No. REC-0107014). Alexandria, Va.: The CNA Corporation. http://www.nbpts.org/UserFiles/File/Final_Study_11204_D_-_Cavalluzzo_-_CNA_Corp.pdf
Cohen, Peter A., James A. Kulik, and Chen-Lin C. Kulik.1982. Educational outcomes of tutoring: A meta-analysis of findings. American Educational Research Journal, 19 (2), Summer: 237–248.
Cooper, Harris, et al. (Barbara Nye, Kelly Charlton, James Lindsay, and Scott Greathouse). 1996. The effects of summer vacation on achievement test scores: A narrative and meta-analytic review. Review of Educational Research, 66 (3), 227-268.
Darling-Hammond, Linda. 2009. Recognizing and enhancing teacher effectiveness. International Journal of Educational and Psychological Assessment, 3, December: 1-24.
Darling-Hammond, Linda. 2010. The Flat World and Education: How America’s Commitment to Equity Will Determine Our Future. New York: Teachers College Press.
Dee, Thomas S. and Brian Jacob. 2009. “The Impact of No Child Left Behind on Student Achievement.” NBER Working Paper No. 15531, November. http://www-personal.umich.edu/~bajacob/files/test-based%20accountability/nclb_final_11_18_2009.pdf; http://www.nber.org/papers/w15531
Diamond, John B., and Kristy Cooper. 2007. The uses of testing data in urban elementary schools: Some lessons from Chicago. Yearbook of the National Society for the Study of Education, 106 (1), April: Chapter 10, 241–263.
Downey, Douglas B., Paul T. von Hippel, and Melanie Hughes. 2008. Are ‘failing’ schools really failing? Using seasonal comparison to evaluate school effectiveness. Sociology of Education, 81, July: 242–270.
Feng, Li, David Figlio, and Tim Sass. 2010. School Accountability and Teacher Mobility. CALDER Working Paper No. 47, June. Washington DC: CALDER. http://www.urban.org/uploadedpdf/1001396-school-accountability.pdf
Finnigan, Kara S., and Betheny Gross. 2007. Do accountability policy sanctions influence teacher motivation? Lessons from Chicago’s low-performing schools. American Educational Research Journal, 44 (3), September: 594-630.
GAO (U.S. Government Accountability Office). 2009. No Child Left Behind Act. Enhancements in the Department of Education’s Review Process Could Improve State Academic Assessments. GAO 09-911. September. http://www.gao.gov/new.items/d09911.pdf
Glass, Gene V. et al. (Leonard S. Cahen, Mary Lee Smith, and Nikola N. Filby). 1982. School Class Size: Research and Policy. Beverly Hills, Calif.: Sage.
Goddard, Yvonne L., Roger D. Goddard, and Megan Tschannen-Moran. 2007. A theoretical and empirical investigation of teacher collaboration for school improvement and student achievement in public elementary schools. Teachers College Record, 109 (4): 877–896.
Goldhaber, Daniel, and Emily Anthony. 2004. Can Teacher Quality be Effectively Assessed? Seattle, Wash.: University of Washington and Washington, D.C.: The Urban Institute. http://www.urban.org/UploadedPDF/410958_NBPTSOutcomes.pdf
Heller, Rafael, Douglas B. Downey, Paul Von Hippel, forthcoming. Gauging the Impact: A Better Measure of School Effectiveness. Quincy, Mass.: The Nellie Mae Foundation.
Hemphill, Clara, and Kim Nauer, et al. (Helen Zelon, Thomas Jacobs, Alessandra Raimondi, Sharon McCloskey and Rajeev Yerneni). 2010. Managing by the Numbers. Empowerment and Accountability in New York City’s Schools. Center for New York City Affairs. The New School. June. http://newschool.edu/milano/nycaffairs/documents/ManagingByTheNumbers_EmpowermentandAccountabilityinNYCSchools.pdf
Hirsch, E.D. Jr. 2006. The Knowledge Deficit. Houghton Mifflin Company.
Hirsch, E.D. Jr. and Robert Pondiscio. 2010. There’s no such thing as a reading test. The American Prospect, 21 (6), July/August. http://www.prospect.org/cs/articles?article=theres_no_such_thing_as_a_reading_test
Jackson, C. Kirabo, and Elias Bruegmann. 2009. “Teaching Students and Teaching Each Other: The Importance of Peer Learning for Teachers.” Cambridge, Mass.: National Bureau of Economic Research, Working Paper No. 15202, August.
Jauhar, Sandeep. 2008. The pitfalls of linking doctors’ pay to performance. The New York Times, September 8. http://www.nytimes.com/2008/09/09/health/09essa.html
Koedel, Cory, and Julian R. Betts. 2007. “Re-Examining the Role of Teacher Quality in the Educational Production Function.” Working Paper #2007-03. Nashville, Tenn.: National Center on Performance Initiatives. http://economics.missouri.edu/working-papers/2007/wp0708_koedel.pdf
Koretz, Daniel. 2008a. Measuring Up. What Educational Testing Really Tells Us. Cambridge, Mass.: Harvard University Press.
Koretz, Daniel. 2008b. (2008, Fall). A measured approach. American Educator, Fall: 18-39. http://www.aft.org/pdfs/americaneducator/fall2008/koretz.pdf
Krueger, Alan B. 2003. Economic considerations and class size. The Economic Journal, 113 (485); F34-F63.
Lazear, Edward P. 1989. Pay equality and industrial policies. Journal of Political Economy, 97 (3), June: 561-80.
Lee, Jaekyung. 2006. Tracking Achievement Gaps and Assessing the Impact of NCLB on the Gaps: An In-Depth Look Into National and State Reading and Math Outcome Trends. Cambridge, Mass.: The Civil Rights Project at Harvard University. http://www.civilrightsproject.ucla.edu/research/esea/nclb_naep_lee.pdf
Lockwood, J. R., et al. (Daniel McCaffrey, Laura S. Hamilton, Brian Stetcher, Vi-Nhuan Le, and Felipe Martinez). 2007. The sensitivity of value-added teacher effect estimates to different mathematics achievement measures. Journal of Educational Measurement, 44 (1), 47 – 67.
McCaffrey, Daniel F., et al. (Daniel Koretz, J. R. Lockwood, and Laura S. Hamilton). 2003. Evaluating Value-Added Models for Teacher Accountability. Santa Monica: RAND Corporation. http://www.rand.org/pubs/monographs/2004/RAND_MG158.pdf
McCaffrey, Daniel F., et al. (J.R. Lockwood, Daniel Koretz, Thomas A. Louis, and Laura Hamilton). 2004. Models for value-added modeling of teacher effects. Journal of Educational and Behavioral Statistics, 29 (1), Spring: 67-101.
McCaffrey, Daniel F., et al. (Tim R. Sass, J. R. Lockwood and Kata Mihaly). 2009. The intertemporal variability of teacher effect estimates. Education Finance and Policy 4, (4), Fall: 572-606.
McMurrer, Jennifer. 2007. Choices, Changes, and Challenges. Curriculum and Instruction in the NCLB Era. July. Washington, D.C.: Center on Education Policy. http://www.cep-dc.org/index.cfm?fuseaction=document.showDocumentByID&nodeID=1&DocumentID=212
McMurrer, Jennifer. 2008. Instructional Time in Elementary Schools. A Closer Look at Changes in Specific Subjects. February. Washington, D.C.: Center on Education Policy. http://www.cep-dc.org/document/docWindow.cfm?fuseaction=document.viewDocument&documentid=234&documentFormatId=3713
Medina, Jennifer. 2010. New diploma standard in New York becomes a multiple-question choice. The New York Times, June 28. http://www.nytimes.com/2010/06/28/education/28regents.html
Milanowski, Anthony T., Steven M. Kimball, and Brad White. 2004. The Relationship Between Standards-based Teacher Evaluation Scores and Student Achievement. University of Wisconsin-Madison: Consortium for Policy Research in Education. http://cpre.wceruw.org/papers/3site_long_TE_SA_AERA04TE.pdf
Mosteller, Frederick. 1995. The Tennessee study of class size in the early school grades. The Future of Children, 5 (2): 113-127
Neal, Derek. 2009. “Designing Incentive Systems for Schools.” In Matthew G. Springer, ed. Performance Incentives. Their Growing Impact on American K-12 Education. Washington, D.C.: Brookings Institution Press.
Newton, Xiaoxia, et al. (Linda Darling-Hammond, Edward Haertel, and Ewart Thomas). Forthcoming. Value-Added Modeling of Teacher Effectiveness: An Exploration of Stability across Models and Contexts.
Packard, Richard and Mary Dereshiwsky. 1991. Final Quantitative Assessment of the Arizona Career Ladder Pilot-Test Project. Flagstaff: Northern Arizona University. http://www.eric.ed.gov/PDFS/ED334148.pdf
Ravitch, Diane, 2003. The Language Police. New York: Knopf.
Ravitch, Diane. 2010. The Death and Life of the Great American School System. Basic Books.
Rothstein, Jesse. 2010. Teacher quality in educational production: Tracking, decay, and student achievement. Quarterly Journal of Economics, 125 (1), January: 175-214.
Rothstein, Richard, Rebecca Jacobsen, and Tamara Wilder. 2008. Grading Education: Getting Accountability Right. Washington, D.C. and New York: Economic Policy Institute and Teachers College Press.
Rubin, Donald B., Elizabeth A. Stuart, and Elaine L. Zanutto. 2004. A potential outcomes view of value-added assessment in education. Journal of Educational and Behavioral Statistics, 29 (1), Spring: 103-116.
Sass, Timothy. 2008. The Stability of Value-Added Measures of Teacher Quality and Implications for Teacher Compensation Policy. Washington, D.C.: CALDER. http://www.urban.org/uploadedpdf/1001266_stabilityofvalue.pdf
Schochet, Peter Z. and Hanley S. Chiang. 2010. Error Rates in Measuring Teacher and School Performance Based on Student Test Score Gains (NCEE 2010-4004). Washington, D.C.: National Center for Education Evaluation and Regional Assistance, Institute of Education Sciences, U.S. Department of Education. http://ies.ed.gov/ncee/pubs/20104004/pdf/20104004.pdf
Smith, Tracy W., et al. (Belita Gordon, Susan A. Colby, and Jianjun Wang). 2005. An Examination of the Relationship of the Depth of Student Learning and National Board Certification Status. Office for Research on Teaching, Appalachian State University. http://www.nbpts.org/UserFiles/File/Applachian_State_study_D_-_Smith.pdf
Solomon, Lewis, et al. (J. Todd White, Donna Cohen and Deborah Woo). 2007. The Effectiveness of the Teacher Advancement Program. National Institute for Excellence in Teaching. http://www.tapsystem.org/pubs/effective_tap07_full.pdf
Van Lier, Piet. 2008. Learning from Ohio’s Best Teachers. Cleveland, Ohio: Ohio Policy Matters, October 7. http://www.policymattersohio.org/pdf/PAR2008.pdf
Vandevoort, Leslie G., Audrey Amrein-Beardsley, and David C. Berliner. 2004. National Board certified teachers and their students’ achievement. Education Policy Analysis Archives, 12 (46), September 8.
Wilson, Mark, and P.J. Hallam. 2006. Using Student Achievement Test Scores as Evidence of External Validity for Indicators of Teacher Quality: Connecticut’s Beginning Educator Support and Training Program. Berkeley, Calif.: University of California at Berkeley.
Authors, each of whom is responsible for this brief as a whole, are listed alphabetically. Correspondence may be addressed to Educ_Prog@epi.org
Eva L. Baker is professor of education at UCLA, co-director of the National Center for Evaluation Standards and Student Testing (CRESST), and co-chaired the committee to revise testing standards of the American Psychological Association, the American Educational Research Association, and the National Council on Measurement in Education.
Paul E. Barton is the former director of the Policy Information Center of the Educational Testing Service and associate director of the National Assessment of Educational Progress.
Linda Darling-Hammond is a professor of education at Stanford University, former president of the American Educational Research Association, and a member of the National Academy of Education.
Edward Haertel is a professor of education at Stanford University, former president of the National Council on Measurement in Education, Chair of the National Research Council’s Board on Testing and Assessment, and a former chair of the committee on methodology of the National Assessment Governing Board.
Helen F. Ladd is professor of Public Policy and Economics at Duke University and president-elect of the Association for Public Policy Analysis and Management.
Robert L. Linn is a distinguished professor emeritus at the University of Colorado, and has served as president of the National Council on Measurement in Education and of the American Educational Research Association, and as chair of the National Research Council’s Board on Testing and Assessment.
Diane Ravitch is a research professor at New York University and historian of American education.
Richard Rothstein is a research associate of the Economic Policy Institute.
Richard J. Shavelson is a professor of education (emeritus) at Stanford University and former president of the American Educational Research Association.
Lorrie A. Shepard is dean and professor, School of Education, University of Colorado at Boulder, a former president of the American Educational Research Association, and the immediate past president of the National Academy of Educatmicigen 2012
by -cvijic boris
Ben Wood
PSM Industrial Mathematics, Michigan State University, 2012
Research Manager – Statistical Methods, Measurement Science, Nielsen
“My desire to solve challenging problems drove me to pursue mathematics; my desire to manage a technical team led me to the PSM. The PSM in Industrial Mathematics at Michigan State University gave me the right experiences to earn a management track position in Data Science, and continues to pay dividends. With a balance of mathematical knowledge, computational experience, and business training provided by the PSM, I am able to solve increasingly challenging mathematical and business problems with a perspective unique among my peers. I am currently managing development of a new behavioral modeling technique at a leading market research firm.”
Bradley W. Bykowicz
PSM Industrial Mathematics, Michigan State University, 2009
Associate Actuary, Corporate Risk Department, Accident Fund
“I graduated in 2009 from Michigan State University with my Master’s of Science in Industrial Mathematics. The MSIM program greatly prepared me for success in industry. Not only did I develop a strong quantitative and analytic background, but I also improved my communication skills and became a well-rounded problem solver. The practical, industry-emphasis of the educational experience provided by the MSIM program made my transition into the working world very easy, and made me a competitive candidate for a number of positions out of school. While I am an actuary by trade, the versatility provided to me by the MSIM experience has allowed me to pursue a number of interesting projects outside of my primary field.”
Camille Archer
PSM Industrial Mathematics, Michigan State University, 2012
Director of Training, Institute for Cyber-Enabled Research (iCER), Michigan State University (MSU)
“I entered the PSM program in Industrial Mathematics after working for three years as a high school mathematics/science teacher – two of which were spent working part-time as an Assessment Manager. In May 2012, I graduated from Michigan State University’s PSM program in Industrial Mathematics and joined the team at L& S Associates Inc. as an Information Manager. I later returned to my alma mater in 2014 to coordinate the high performance computing education and training efforts at MSU’s iCER. Many of the communication and collaboration skills I use today I learned through my exposure as a graduate student to collaborative class and industry projects as well as the certificate program in Project Management. The later being a co-requisite for graduation from the PSMIM program.
In addition to a solid mathematics component, the flexibility of the program allowed me to pursue my interests in statistics and data mining, which were very useful in my role as an Information Manager. I can truly say that the academic and professional education I received through the PSM program in Industrial Mathematics at MSU broadened my career prospects.”
Elizabeth Carter
PSM Industrial Mathematics, Michigan State University, 2013
Senior Staff Game Designer, WMS Gaming
“I graduated from Michigan State University’s PSM program in Industrial Mathematics in May 2013 and started at WMS Gaming in October 2013. I joined the PSM program because it offers a unique blend of pure mathematics, applied mathematics, and project management, with a strong emphasis on communication and personal professional development. Throughout the program students learn useful skills via the courses but they also learn how to create a strong projection of themselves, which is often something both young and experienced professionals are lacking. Much of my job entails communicating with foreign studios, so being able to concisely summarize all of the relevant information into a single email helps cut down on time lost due to the time difference and language barrier. The program also helps students develop confidence in their public speaking and presenting skills, which is useful in almost any employment setting.”
Chad Stripling
PSM Industrial Mathematics; Michigan State University, 2014
Data Analyst, Dunnhumby
“Coming out of my undergraduate studies I had several internships and research experiences that helped me decide that I wanted to use my mathematical background in a business setting. I did not know however what type of opportunities there was for a mathematically minded person who wanted to work in industry. The Industrial Mathematics program at Michigan State not only allowed me to continue to develop my mathematical and business acumen but also allowed me to gain valuable work experience. I was able to work with companies and solve real business needs as part of the program which was extremely helpful in better understanding what I wanted to do for a career and finding a job upon completion of the program. The experiences I had and the knowledge I gained during my time in the Industrial Mathematics program gave me a strong foundation to start my career and has helped set me apart from my peers while beginning my career in marketing and customer science analytics. The Industrial Mathematics program at Michigan State was one of the best decisions I have ever made in terms of growing as mathematician and business professional, while gaining the background necessary to be successful in the marketplace.”
Cody Dean
PSM Industrial Mathematics, Michigan State University, 2010
Sr. Business Intelligence Analyst, Amway Corporation
“I graduated from Michigan State University’s PSM program in Industrial Mathematics in May 2010 and joined Amway in July 2010. The group that I came into at Amway had physicists, electrical and computer engineers, and bio-chemists, to name a few, and needless to say the group was extremely diverse. By allowing me to take graduate level classes in so many different disciplines, the Industrial Mathematics program made me a very well-rounded employee capable of collaborating with these individuals right out of the gate. I was able to effectively communicate with each discipline and the learning curve to get caught up to speed was quite low. Now that I have been with the company for 5 years, the mathematical and statistical background that I developed at MSU has put me in a role where people routinely seek me out for my expertise. I think one of the most beneficial pieces of the program was the business training that we received. I can effectively communicate my ideas to others in different areas of the company and I am constantly praised for my communication and presentation abilities. My experience in the Industrial Mathematics program at MSU helped me land a career that I absolutely love.”
Cody Stripling
PSM Industrial mathematics, Michigan State University, 2012
Senior Analyst, Dunnhumby
“Michigan State University’s Professional Science Master’s program in Industrial Mathematics allowed me the opportunity to gain a solid foundation in mathematics, statistics, and data analysis before beginning my career. I graduated in 2010 and began my job at dunnhumby as an analyst. My breadth of previous experience and work has helped grow in my position where I analyze, interpret, model, and manipulate dat. The classes and real world projects that were available to me because of the PSM program provided a great basis for the challenges I come across in my day to day work.”
Curt Baragar
PSM Industrial Mathematics, Michigan State University, 2006
Mathematics Instructor, GRCC
“After graduating from MSU’s Industrial Mathematics program I was working as a consulting engineer at a telecommunications firm. Later I accepted an offer for a teaching position at a local community college. The wide variety of applied mathematics from various fields that I was exposed to in the PSM program gave me a great appreciation for the seemingly endless types of problems that are being solved using mathematics. This, along with the real-world experience gained from our projects class, makes it very easy for me to answer the inevitable student question, ‘When are we ever going to use this?’ ”
Dmitry Mikhaylov
PSM Industrial Mathematics, Michigan State University, 2014
“Obtaining PSM in Industrial Mathematics at Michigan State has been a very beneficial experience. First, the program offered a big variety of cognates. I took courses in statistics, marketing, and entrepreneurship. Other options included engineering, supply chain, and computer science. Second, one of the program’s requirement was a participation in the research project for local industries. This appeared to be very beneficial as I was able to immediately apply my newly obtained knowledge and skills. Finally, the program paid a special attention to teamwork and professionalism, both of which are necessary in any business environment. I would definitely recommend applying to this program for those who look to deepen their academic background, but at the same time are focused on the careers in business.”
Ian Rhynard
PSM Industrial Mathematics, Michigan State University, 2012
Emerging Leaders Associate, Nielsen
“I always loved mathematics but as I followed my passion in college I struggled to find ways to apply it in my day to day work life. The Professional Science Master’s in Industrial Mathematics at Michigan State University provided me with the computational tools and professional experience that I needed to excel in industry and apply those skills on a daily basis. This program has provided me with opportunities in a variety of areas including mechanical engineering, data mining, and supply chain management that I otherwise would not have been qualified for. Everyone who wants to apply mathematics in their profession needs to consider this program as a critical stepping stone towards their career.”
Ina Stashonsky
PSM Industrial Mathematics, Michigan State University, 2010
Anesthesiologist Assistant, Grady Memorial Hospital
“After graduating from the Professional Science Master’s (PSM) degree program in Industrial Mathematics, I began my career at Deloitte & Touche, LLP as a consultant. Specifically, I worked in the IT audit department analyzing figures and reports produced by my client. The skills obtained in the PSM program were useful to my profession because of the programming that was required and the type of analysis used to test samples from a large data base. In addition, I was prepared with a strong work ethic and ability to communicate well with others.
My career path took a turn, as I chose to seek a profession as an anesthesiologist assistant. Even though my ‘office’ is now the operating room, I have been easily able to transfer the skills obtained from the PSM program. Attention to detail and analysis – as was emphasized in the PSM Industrial Mathematics program – is critical to a successful outcome in both professions. My background is different from many of my colleagues but I see that as an asset to the anesthesia team and am able to contribute my unique skills each day at work.”
James F. Kelly
PSM Industrial Mathematics, Michigan State University, 2005
Sr. Software Engineer – Simulation Group, Exa Corporation
“I graduated from MSU’s PSM program in Industrial Mathematics in August 2005 and stayed at MSU to complete a Ph.D. In Electrical and Computer Engineering in December 2008; nevertheless, I consider myself at Applied Mathematician at heart and have continued to learn and publish Applied Mathematics since leaving MSU. Mathematics and Physical Science is a journey I began in high school, a journey I continued as an undergraduate at Penn State and as a graduate student at MSU and later as postdoc at the Naval Postgraduate School (Monterey, CA) and now at my current position in industry at Exa Corp. (Burlington, MA), where we develop computational fluid dynamics (CFD) software for the automotive, oil and gas, and aerospace industries. The courses, projects, and conversations I had during the Industrial Math program provided the foundation for this journey. There were some sharp people at MSU; not only sharp, but sharing, and compassionate, and human. I’ve stayed in contact with several of my classmates, and seen them take different paths through life.
Mathematics gives you the tools to predict, to question, and to test your assumptions. Everyday I learn something knew. For instance, yesterday, I learned how to express three-dimensional rotations in terms of a matrix exponential, allowing one to express reference frame transformations in multiply nested reference frames. This sounds esoteric, but the auto companies want to simulation rotating tires on a car that banks around a corner. Basic mechanics combined with high performance computing gives us the tools to tackle this problem. I’ve also worked on numerical weather prediction problems, and learned a little about climate change. The common language is math; the common attitude is scientific doubt.
I also learned something about teamwork in the PSM program. We all have different strengths, different limitations. The problems we face are difficult, but they are surmountable. We have to work together, to respect each other, to contribute in our way. We have to listen, and work together with a community of like-minded people. It’s been almost twelve years since I stepped off the Amtrak in East Lansing on August 15, 2003, but this is what I learned, and am continuing to learn.”
Jinxuan Yan
PSM in Industrial Mathematics, Michigan State University, 2013
Data Analyst, PCS-CTS
“It was a life-changing experience to join the PSM in Industrial Mathematics from MSU. It was totally new environment, in which I had the opportunity to take classes from the top and brilliant professors from different departments, Math, Statistics and Economic, Engineering and so on. And the most exciting thing is that I can participate in all kinds of social events to meet the industrial advisors and build my interview skills and gain valuable insights into the fields. The Industrial projects gave me a chance to gain some real industrial experiences, which has prepared me as a team player and also as a leader. With the diverse knowledge in Applied Math, Statistics and data mining and training in effective communication and leadership, I am very well prepared for my career.”
Jun Wang
PSM Industrial Mathematics, Michigan State University, 2004
Data Scientist, Twitter
“After graduating from Michigan State University’s PSM program in Industrial Mathematics in December 2004, I joined HSBC’s Risk Management department working on loan pricing and portfolio management strategies. In 2007 I joined Google, in the 5 years following I worked in different products: business analytics, operations, marketing and business intelligence and built multiple teams along the way. Now I’m at Twitter’s revenue team working on Sales Intelligence using data science. I’m sure my professional career would be completely different if I were not so well prepared by the PSM program for the industry world. During my two years in the PSM program I had the opportunity to explore my interest in a wide range of topics from economics to data mining, from bio-stats to computer science. In additional to the wide academic offerings, I also acquired enough knowledge through the certificate program on real world business skills around project management, presentation, writing, etc. — all happening while surrounded by a group of very talented, ambitious and diverse group of students. As a student who had almost exclusively focused on academic math and near zero exposure to the business world before, the PSM degree was a major booster in both my business acumen and my self-confidence.”
Kaylee Linthicum
PSM Industrial Mathematics, Michigan State University, 2011
Applied Mathematician, Supplier Management Finance, The Boeing Company
“I joined the Michigan State University PSM program in Industrial Mathematics after earning a Bachelor’s in Computational Mathematics. In addition to coursework that strengthened my core knowledge of applied mathematics, statistics, programming, and data mining, the program offered experiences with local companies which allowed me to practice these skills on real world problems. There was also a focus on clear communication which has benefitted me greatly in my career, especially when explaining technical data to non-technical colleagues. After graduating, I joined Boeing as a finance analyst in the Supplier Management Finance organization and have recently transitioned into a role as an applied mathematician working on cost models for the same organization. Throughout my time here, the skills that I developed in the PSM in Industrial Mathematics program have been recognized and allowed me opportunities to contribute to the success of my organization. As a part of the program, I participated in a Business and Communication Certificate program which exposed me to topics related to business which I have been able to build on as I spend more time in the business environment.”
Luma Vasiljevic
PSM Industrial Mathematics, Michigan State University, 2006
Assistant Manager – Network Optimization Transportation Planning, Norfolk Southern Corporation
“I graduated from Michigan State University’s PSM program in Industrial Mathematics in December 2006 and joined Norfolk Southern Corporation in January 2007. I was impressed by the quality and diversity of the students attracted to the PSM program. Having a strong background in theoretical mathematics, the program helped me strengthen my mathematical modeling and programming skills and exposed me to a variety of real world problems where mathematical modeling is applicable. The program also helped me improve my communication and writing skills. I believe that the skills and knowledge acquired through the PSM program lie at the core of my career success thus far; However, I believe that exposure to organizational structures and decision making processes as well as organizational data structures and actual data during the program would have eased the transition from academia to industry and made me even more effective in my role.”
Matt Kowske
PSM Industrial Mathematics, Michigan State University, 2008
Senior Consultant, Operations Excellence – West Monroe Partners
Senior Solution Consultant, Manufacturing – Kronos Inc.
LEAN 6-Sigma Facility Manager, 3PL – Caterpillar Logistics
Distribution Supervisor, 3PL – Caterpillar Logistics
I graduated from the Industrial Mathematics PSM program from MSU the summer of 2008 and was recruited to a management rotational program with Caterpillar Logistics. Being a world leader in manufacturing as well as logistics, Caterpillar always searches for well-rounded and analytical talent. The PSMIM program at MSU was pivotal in providing this diverse background to take my career to the next level. The program allowed me to better understand business specific analysis; how to tailor deliverables to a specific audience; and how to communicate in a succinct way. These were critical in the next steps of my career working in a consulting capacity; firstly by designing time and labor tracking solutions for manufacturing customers across the world, and most currently by providing management consulting with a focus on customers’ operational excellence. Below are just some of the experiences I have been able to have with the help of the PSM in Industrial Mathematics program.
Functional/technical experience:
|
Industry Experience:
|
Education:
|
Certifications:
|
Megan Baker
PSM Industrial Mathematics, Michigan State University, 2013
Actuarial Analyst, Accident Fund
“I graduated from Michigan State University’s PSM in Industrial Mathematics in May 2013. This program allowed me to strengthen my communication and writing skills. I was also able to experience mathematics in more real world scenarios, including experience with local companies in projects outside of the classroom. I believe the knowledge and experience I gained from this program have allowed me to be even more successful in my career.”
Nandita Singh
PSM Industrial Mathematics, Michigan State University, 2005
Senior Manager, Customer Experience Design & Operations Strategy – Discover Financial Services
“PSM in Industrial Mathematics program prepared me to step in the corporate world where Mathematical and Statistical concepts can be applied to solve business problems. The curriculum also included project work with a Company and certification in communication skills, which immensely helped me to be successful in my career right from the beginning. Since graduation, I have been working for Financial Services Firm with focus on Customer experience, Strategic Operations, Marketing Analytics, and Risk Management.”
Ryan Bettinger
PSM Industrial Mathematics, Michigan State University, 2011
Earned Value/Cost Analyst, Tecolote Research, Inc.
“I graduated from the Michigan State University PSM program in Industrial Mathematics in May 2011 and joined Tecolote Research, Inc. in October 2011. The program provided the correct balance of technical prowess and business acumen allowing me to transition quickly to the field of cost analysis. The ability to translate engineering projects and designs to understandable concepts and ideas for business decision makers is fundamental to the success of any program.
The MSU PSM program demands that its graduates are accomplished technical, programmatic and effective assets to their chosen fields. The flexibility of the program permits these goals to be met while allowing all candidates to mold their studies to future desired career paths without unnecessarily pigeonholing graduates to a specific job or vocation. My successes have been greatly amplified by the training provided through the MSU PSM program. There is no comparison for the combination of technical aptitude and common business sense that is provided and learned through the course of study.”
Tarah Jensen
PSM Industrial Mathematics, Michigan State University, 2012
“After completing my bachelor’s degree in Mathematics from Grand Valley State University, I was looking to further my education in mathematics. I desired a program that would challenge me, allow me to take a diverse group of classes, and give me resources to apply my data analysis skills in real situations. I found this program at Michigan State. As a student in the Industrial Mathematics program, I could incorporate courses in other departments, such as business and marketing, with my math courses. Seeing issues from different perspectives pushed me to be better at finding solutions to problems. In my first year, I was involved in a challenging research project with Ford Motor Credit Company. Through this project, I further developed skills that I even use to this day. As an analyst for a charter school organization, I am constantly analyzing data in new ways to provide stakeholders with important information. I know my time in the Industrial Mathematics program at Michigan State has helped me to be successful in the work I do.”
Yunyun Wei
PSM Industrial Mathematics, Michigan State University, 2014
Software Engineer, ForteMedia, Inc.
“I am passionate about science and the disciplines of business. With the career goal of being a business and science professional in a technology-based company, right after I finished my undergraduate study in Physics in 2012, I joined the PSM in Industrial Mathematics program at Michigan State University (MSU).
During the two years’ graduate school life in the Industrial Mathematics program at MSU, I learned, worked and grew up with an inspiring advisor and an amazing group of teammates in this program. I came to work in the Bay Area, CA after I graduated. The exposure to project management during the program enables me to transit from academia to industry very quickly. In addition to technical knowledge, the work skills, particularly communication and writing skills, and the ability of presenting professionalism I acquired from this program are valuable in my work. I believe those are essential for one to keep growing in one’s career.”
Zhongping Gao
PSM Industrial Mathematics, Michigan State University, 2011
Manager – Model Risk, Santander Bank Holding Company
“After joining the industrial mathematics program in 2009, I had invaluable experience to learn using statistics, finance, and mathematics to solve real industrial problems. Thanks to the great connection with industrial companies, I worked and learnt closely with industrial professionals on multiple projects. My career directly benefits from the mathematical skills acquired from this program. As a model risk manager at Santander bank, I am leading a team to review all model risk related projects for capital planning process, and to response regulator’s examination, which require exactly the strong mathematical and analytic skills leant from PSM. I believe that what this program offered is great combined knowledge between theory and application.”
Department of Mathematics
Michigan State University
619 Red Cedar Road
C212 Wells Hall
East Lansing, MI 48824
Phone: (517) 353-0844
Fax: (517) 432-1562
Worldwide B2B and e-commerce. From traditional country products to micro businesses and exclusive products. Minimalist design – extreme ease of use – targeting people from 15 to 80̵… Izvor: Work at…
Izvor: Work at BUYBYCOUNTRY
Enter a caption more videos PowerBatt Global presence and recognition Presence on 4 continents and 28 countries In co-operation with Pilsen Science and Technology…
Izvor: PowerBat
PowerBatt
PowerBatt
16. 08. 2016PowerBatt Golf carts 14 months ago, at the beginning of 2015, we have started a pilot test in Ciputra Golf resort, Surabaya…
PowerBatt brings to its clients significant savings up to 85% in comparison with purchasing of new battery!
PowerBatt represents preventive and sensitive solution for treatment of lead-acid batteries with the long-term effects.
Regular use of PowerBatt is currently the easiest and the most effective way of reducing the costs related to the battery management.
At the same time it has positive environmental effects as well.
Worldwide B2B and e-commerce. From traditional country products to micro businesses and exclusive products. Minimalist design – extreme ease of use – targeting people from 15 to 80̵…
Izvor: Work at BUYBYCOUNTRY
Worldwide B2B and e-commerce. From traditional country products to micro businesses and exclusive products. Minimalist design – extreme ease of use – targeting people from 15 to 80’s. Five sources of income in one global site. PPL, Commissions on Direct Sales, Advertising,Commission on Micro Company Sales, Profit on countryXclusive products. Send traditional gold filigree earrings from Portugal to Suriname. Buy Robert Mondavi wines from USA and have a case delivered to Christchurch in New Zealand. Buy an amber necklace from Lithuania and have it delivered to Nigel, South Africa. Every country has a “Countrypreneur” making sure that products are delivered timeously. Countrypreneurs also source rare merchandise from their Country to be sold exclusively on BuyByCountry. We own both BUYBYCOUNTRY and BYEBYECOUNTRY. Finally …a website where you can get accurate results for what you are looking for. Italian short boots? Italy>Shoes>Boots an
d only Italian boots will be displayed.
Worldwide B2B and e-commerce. From traditional country products to micro businesses and exclusive products.
Minimalist design – extreme ease of use – targeting people from 15 to 80’s.
Five sources of income in one global site. PPL, Commissions on Direct Sales, Advertising,Commission on Micro Company Sales, Profit on countryXclusive products.
Send traditional gold filigree earrings from Portugal to Suriname. Buy Robert Mondavi wines from USA and have a case delivered to Christchurch in New Zealand. Buy an amber necklace from Lithuania and have it delivered to Nigel, South Africa.
Every country has a “Countrypreneur” making sure that products are delivered timeously. Countrypreneurs also source rare merchandise from their Country to be sold exclusively on BuyByCountry.
We own both BUYBYCOUNTRY and BYEBYECOUNTRY.
Finally …a website where you can get accurate results for what you are looking for. Italian short boots? Italy>Shoes>Boots and only Italian boots will be displayed.
THE NEXT STEP TOWARDS EARLIER ACCESS TO DRUGS IN DEVELOPMENT Born out of personal experience, we felt there was something inherently wrong in the rationale how access to investigational drugs for p…
Izvor: myTumorrows
THE NEXT STEP TOWARDS EARLIER ACCESS TO DRUGS IN DEVELOPMENT |
||||
Born out of personal experience, we felt there was something inherently wrong in the rationale how access to investigational drugs for patients without treatment options was organized. I would have expected that being a medical doctor and having led a large pharma company would have helped me in navigating local approval, regulatory affairs and discussions with biotech back in 2012. This was not the case. Read more → |
||||
Written by |
||||
|
|
Born out of personal experience, we felt there was something inherently wrong in the rationale how access to investigational drugs for patients without treatment options was organized. I would have expected that being a medical doctor and having led a large pharma company would have helped me in navigating local approval, regulatory affairs and discussions with biotech back in 2012. This was not the case.
It was then that myTomorrows was born. myTomorrows aims to make development stage drugs available to physicians and their patients facing unmet medical needs, those who cannot participate in clinical trials due to the exclusion criteria.
Combining deep medical, technological and regulatory expertise, we have proven that the platform model for facilitating drugs in development works — for physicians and their patients, for drug makers, and even for governments. Over the last years we have been able to support hundreds of physicians in many countries in treating patients that would otherwise have not had any further options. Where patients are facing an unmet medical need, we see our role in breaking down barriers and making access to promising drugs possible.
Last week we announced that two new investors, Octopus Ventures from London and EQT Ventures from Stockholm, have joined myTomorrows. Together with Balderton Capital and Sofinnova Parters, who supported us already in 2014, this group invested €10m in myTomorrows. They share the belief that the current model for access to development-stage medicines is there because of tradition rather than because it puts the patient first or helps in gathering the best data to practice rational pharmacotherapy. There is a real problem that needs to be solved and with the support of a strong and experienced team, this is the next step towards change.
With the necessary funding in place, we want to attract key multidisciplinary talent that can help us to further unburden regulators and turn the traditional access model on its head. Physicians and patients can expect that myTomorrows will become active in more countries to help more patients globally.
The way medicines are developed today is changing. We will see the collection of efficacy data move out of an artificial clinical setting and into the real world, treating regular patients. Focusing on creating access for the large group of patients that cannot participate in clinical trials, the myTomorrows concept will play a key role in the development of real-world evidence collection.
Alongside the funding round to help expand the myTomorrows vision, James Shannon has been appointed chairman of the supervisory board. I am personally very happy to have someone like James head up the myTomorrows board, with his heavy experience as Chief Medical Officer at GlaxoSmithKline as well as leading pharma development at Novartis he is a very inspiring man. In his words, this is what he believes myTomorrows will mean to physicians and their patients in future:
“Signaling regarding drug effectiveness will increasingly move to the real world setting with data sets coming from a wider range of patients than are normally included in typical clinical trials. myTomorrows changes the drug development model as we know it, for the better. The technology layers make searching and accessing investigational drugs a seamless process for physicians and their patients,”
— James Shannon MD.
Expanding access to drugs in development. We provide services to patients and physicians in need of diagnostic tests and drugs in development.
Facilitating services to physicians and patients in need of drugs in development when there are no treatment options left.